Fabled Route-Scouters Riding AI Applications

By Louis Garguilo, Chief Editor, Outsourced Pharma
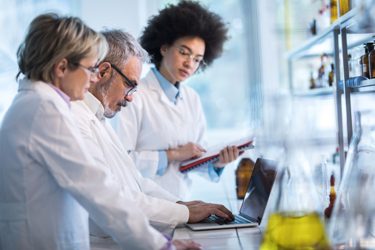
I’ve always had a place in my heart for the route scouters – those self-effacing hunters of small-molecule simplicity, searching for efficient pathways to commercial success, fearless in pointing out risks, and at times, dead ends.
These professionals operate unassumingly between the often better acknowledged medicinal chemists (“We synthesized it!”) and the API folks (“We got the batches!”).
And they have faced a mounting challenge.
Over the last two decades, the complexity of small-molecule APIs has increased on average from eight to 20 synthetic steps.
At times, drug candidate programs arrive at the feet of chemists with numbers of steps that years ago would have disqualified the drug candidate outright.
But now, can the shining star of artificial intelligence (AI) bring more clairvoyance to these battered scientists?
Particularly, the application of AI to process development has filtered into your CDMOs, potentially enabling more of your drug candidates to become viable – and profitable – drugs.
CDMOs Go AI
On the front end of the drug/therapy life cycle, I’m certain readers are familiar with (and your organization is using) AI as a part of your data-acquiring strategies in research and discovery.
Earlier this year I spoke at length with AI-and-pharma subject matter expert Professor Tinglong Dai of Johns Hopkins on the theme of AI in manufacturing settings.
That’s where Elsevier comes in, says Swienty-Busch. The company has created Reaxys, a chemistry database with “a billion-plus chemistry facts” enhanced by its AI partner, Pending.AI.
Swienty-Busch says Reaxys is a “data-driven tool to assist process chemists in utilizing AI to pinpoint more efficient and shorter synthetic routes.”
Earlier this year, the CDMO utility was documented in a collaboration between Elsevier and Lonza that zeroed in on route scouting.
I arranged a discussion with Swienty-Busch of Elsevier (who participated from Germany), and for Lonza, Dr. Simon Wagschal, Associate Director, Small Molecules R&D (Switzerland), and Aaron Johnson, Cheminformatics Data Scientist, Small Molecules R&D (Bend, Oregon).
Wagschal set the stage: Because of this continuously increasing complexity of candidates, he says, “We process chemists can no longer simply refine the med-chem route to create commercially viable synthetic routes.”
To address this, chemists can now actively leverage innovative technologies in the route design process. This, says Wagschal, includes a combining of his organization’s proprietary informatics, advanced automation technologies, and now AI.
As alluded to above, for some years, AI in our industry has been focused on discovery and research – mushrooming databases enabled by advancing AI algorithms and simulations to help find candidates to pursue.
In fact, telling enough, Elsevier’s website for Reaxys describes the product as “… supporting innovation in drug discovery, chemical R&D and academia.”
The door for AI into development, though, is now wide open.
Retro-Synthetic Tools
Johnson, a cheminformatics data scientist, says, “I would agree there have been very clear favorites for implementation of AI in biopharma.”
“Process chemistry, scaling up, or other development activities have not been the biggest topic, especially if you look in the academic literature, or look at how AI has actually been utilized so far.
“But what we are seeing could be described as the next wave of interest in AI.
Particular to the context of CDMOs, we are now and look forward to taking advantage of these shifts.”
This, Johnson says, is part of and largely driven by investments in digitalization, and the overall pushing for Industry 4.0.
“Yes, these retro-synthetic tools still have their limits,” he explains, using a term he’s fond of. They're extremely powerful, but they were predominantly designed with the discovery chemist in mind.
“The AI works backwards until it can find a starting material. These starting material libraries are very discovery-chemistry biased.”
To counter that limitation, both Johnson and Wagschal point to how their organization has integrated its proprietary supply-chain database – containing information of cost, availability, material producer – with AI to essentially allow them to propose routes that are “more supply-chain aware.”
“Not only do we consider a route,” says Wagschal, “we can consider the availability, pricing fluctuations, and parameters on thousands of relevant chemical building blocks.”
“We compare chemical routes, and propose ones that we have a higher confidence is viable from a supply-chain perspective. This allows us to tell our customers, ‘Hey, we can reliably scale up this reaction.’”
He adds: “As we've talked with customers, it's clear there's a significant need for flexible manufacturing solutions to overcome challenges like regulatory hurdles and the development of these very complex APIs we see today.”
Wagschal believes that fundamentally, perhaps the greatest difference now as compared to the past for client relationships is chemists at the CDMO have vastly improved “raw material analysis via AI application.”
But this advancement has needed more than adding a dose of AI to current operations.
“Through our own internal investigations,” says Johnson, “we’ve found that when we use these AI-enabled retro-synthetic tools such as Reaxys, our already capable SMEs are often able to create even shorter routes.”
Data From The 18th Century
Back to Swienty-Busch, he says Elsevier has evolved from its early days as medical publisher. “We see ourselves as a provider of information-based analytics and decision tools for our customers, including CDMOs,” he says.
“We’ve been collecting chemical information since the late 18th century in a huge repository of chemistry data.”
He explains that in 2015 some academic researchers had ideas on applying neural network technologies and modern search methods to create synthesis plans.
Subsequently, in 2018, Marwin Segler and Mark Waller published a paper that “was a game-changer in the field of predictive retrosynthesis,” avers Swienty-Busch.
“Mark Waller formed a company, Pending.AI, based in Australia. We partnered with them to integrate their predictive solution in Reaxys.”
Swienty-Busch’s is not the only advancing AI software, and other CDMOs I talk to are also actively incorporating and adapting to the new technology.
So there’s an AI revolution of sorts at CDMOs, and in the middle of the drug life cycle. In part 2, we learn how this is generating a new generation of route scouters.