Upskilling Your Quality Team For The AI Revolution In Pharma 4.0
By Erika Roberts, ELR Lab Services LLC
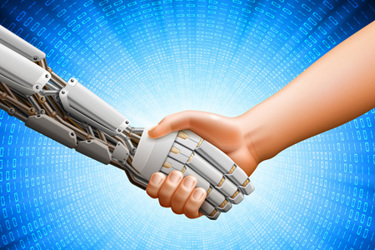
The pharmaceutical industry is undergoing a seismic shift, driven by the integration of artificial intelligence (AI) into manufacturing and quality control processes. This transition, often referred to as Pharma 4.0, will lead to a fundamental evolution in the skillsets of quality assurance and control personnel. While advanced AI systems offer unprecedented capabilities for data analysis, process optimization, and predictive quality assessments, their efficacy is contingent upon the proficiency of the individuals who interact with and interpret their outputs.
A large gap potentially exists between the rapid advancement of AI technologies and the current training programs designed for quality staff. Traditional training methodologies, primarily focused on the rote memorization of standard operating procedures (SOPs) and manual process execution, are insufficient to prepare personnel for the complexities of AI-driven quality systems. This disparity poses a significant risk to the integrity and reliability of pharmaceutical manufacturing processes.
The integration of AI into quality introduces a move away from reactive, event-driven quality management to proactive, data-centric methodologies. This transition requires personnel to possess a nuanced understanding of AI algorithms, data analytics, and the application of these tools to the quality department. Without adequate training, quality staff may struggle to effectively interpret AI-generated insights, leading to potential misinterpretations, flawed decision-making, and compromised product quality.
The impending skills deficit represents a systemic challenge that must be addressed to ensure the successful implementation of Pharma 4.0. The ability to leverage AI for quality is not merely a matter of technological adoption but a cultural and educational transformation. The pharmaceutical industry must prioritize the development of comprehensive training programs that equip quality staff with the information and technical skills necessary to navigate the complexities of AI-integrated quality systems. Failure to address this skills gap will result in lower productivity, increased risk of noncompliance, product deviations, and, ultimately, a compromise in patient safety.
The Power Of AI And Natural Language Model (NLM) Prompt Engineering In Quality Control
The integration of AI and natural language model (NLM) prompt engineering into pharmaceutical quality departments represents a significant advancement in data analysis and process optimization. AI algorithms, particularly machine learning models, are capable of processing vast data sets with unparalleled speed and accuracy, identifying patterns and anomalies that may elude human observation. This capability is particularly valuable in the context of pharmaceutical manufacturing, where meticulous data analysis is essential for ensuring product quality and regulatory compliance.
Specifically, AI can:
- Automate Data Analysis: AI algorithms can autonomously analyze large volumes of process data, identifying trends, deviations, and potential quality issues in real time. This reduces the reliance on manual data review, minimizing the risk of human error and enhancing the efficiency of quality control processes.
- Perform Predictive Quality Assessments: Machine learning models can be trained to predict potential quality deviations based on historical data and process parameters. This proactive approach allows for early intervention, preventing costly product recalls and minimizing disruptions to manufacturing operations.
- Conduct Intelligent Deviation Investigations: AI can assist in the investigation of quality deviations by analyzing relevant data and identifying potential root causes. This accelerates the investigation process, enabling rapid corrective and preventive actions (CAPA).
Furthermore, NLM prompt engineering plays a crucial role in facilitating effective human-AI interaction. By crafting precise and well-structured prompts, quality staff can effectively communicate with AI systems, extracting relevant information and generating actionable insights. This capability is particularly important for tasks such as:
- Data Retrieval and Summarization: NLMs can be used to retrieve and summarize relevant information from large data sets, enabling quality staff to quickly access critical insights.
- Report Generation: NLMs can automate the generation of quality control reports, ensuring consistency and accuracy.
- Knowledge Base Interaction: NLMs can facilitate access to and interaction with knowledge bases, enabling quality staff to quickly access relevant information and best practices.
The synergistic application of AI and NLM prompt engineering empowers quality staff to leverage data-driven insights, enhance process efficiency, and ensure the consistent production of high-quality pharmaceutical products.
The Skills Gap: Why Traditional Training Methods Are Becoming Obsolete
The pharmaceutical industry's reliance on traditional training methodologies, characterized by a focus on the memorization of SOPs and the execution of manual processes, presents a significant impediment to the effective integration of AI-driven quality systems. These conventional approaches, while adequate for historical manufacturing paradigms, do not address the cognitive and analytical demands of Pharma 4.0.
The fundamental limitations of traditional training programs can include:
- Static Knowledge Transfer: SOP-centric training primarily focuses on the transfer of static, predefined knowledge. This approach does not foster the development of critical thinking, problem-solving, and data analysis skills necessary for navigating the dynamic and data-intensive environment of AI-integrated quality control.
- Lack of AI Familiarity: Traditional training programs typically lack comprehensive instruction on AI principles, algorithms, and applications in pharmaceutical quality. This deficiency leaves quality staff ill-equipped to understand and interact with AI systems, hindering their ability to leverage AI-generated insights.
- Insufficient Data Literacy: The increasing reliance on data-driven decision-making in Pharma 4.0 necessitates a high level of data literacy among quality staff. Traditional training methods often fail to provide adequate instruction on data analysis, interpretation, and visualization, limiting the ability of personnel to extract meaningful insights from AI-generated data.
- Inadequate Prompt Engineering Training: Effective interaction with NLM models requires specific skillsets related to prompt engineering. Traditional training programs are lacking in this area, which directly impacts the ability of quality personnel to effectively use AI systems.
As the phasing out of traditional training methods happens with the ushering in of newer technologies, it underscores the urgent need for a greater shift in pharmaceutical education now. The industry should embrace a more dynamic and comprehensive approach that emphasizes critical thinking, data literacy, AI fluency, and continuous learning. If companies do not address this skills gap, it could impede the successful implementation of Pharma 4.0.
A Road Map For Integrating AI And NLM Training
The successful integration of AI and NLM technologies into pharmaceutical quality systems necessitates a systematic and comprehensive approach to training. The following road map could serve as a starting point for developing and implementing an effective AI and NLM training program:
- Assessment
- Conduct a thorough assessment of existing quality staff competencies, identifying gaps in AI knowledge, data literacy, and prompt engineering skills.
- Evaluate the current state of AI integration within the organization, determining the specific AI applications and NLM tools that require training.
- Analyze future AI integration plans to anticipate evolving training needs.
- Curriculum Development
Design a modular training program that encompasses:- AI Fundamentals: introduction to AI principles, machine learning algorithms, and applications in pharmaceutical quality
- NLM Prompt Engineering: techniques for crafting effective prompts, interacting with NLM models, and extracting relevant information
- Data Analysis and Interpretation: instruction on data visualization, statistical analysis, and the interpretation of AI-generated insights
- Problem-Solving and Critical Thinking: development of skills for applying AI insights to quality investigations and decision-making
- Hands-on Exercises and Simulations: practical application of AI and NLM tools through real-world scenarios and simulations
- Develop training materials that are aligned with regulatory requirements and industry best practices
- Implementation
- Use a blended learning approach, combining online modules, workshops, and simulations to cater to diverse learning styles.
- Integrate AI-powered learning platforms to provide personalized training and track individual progress.
- Establish mentorship programs and communities of practice to facilitate knowledge sharing and peer support.
- Provide access to relevant AI and NLM tools, enabling hands-on practice and real-world application.
- Evaluation and Continuous Improvement
- Establish clear metrics to evaluate the effectiveness of the training program, including knowledge assessments, performance evaluations, and feedback surveys.
- Regularly update the training curriculum to reflect advancements in AI technologies and evolving industry needs.
- Implement a continuous feedback loop to gather input from trainees and stakeholders, ensuring the program remains relevant and effective.
This structured approach will help to ensure that quality staff are equipped with the necessary skills to effectively leverage AI and NLM technologies, contributing to the successful implementation of Pharma 4.0.
The Future Of Quality: Embracing The AI-Powered Workforce In Pharma 4.0
The trajectory of pharmaceutical quality departments is linked to the continued evolution of artificial intelligence, particularly within the framework of Pharma 4.0. As AI technologies become increasingly sophisticated and integrated into manufacturing processes, the role of quality staff will undergo a profound transformation within this new industrial process. The future of quality in Pharma 4.0 will be defined by a symbiotic relationship between human expertise and AI capabilities.
The AI-powered workforce within Pharma 4.0 will not replace humans but rather augment it. Quality staff will retain their critical thinking, problem-solving, and ethical judgment, while AI systems will provide the computational power and data analysis capabilities necessary to enhance efficiency and accuracy within the interconnected systems of Pharma 4.0.
This future vision will need to move toward an organizational culture within the Pharma 4.0 framework that fosters a collaborative environment where humans and AI work together. Pharmaceutical companies must prioritize the development of AI-literate leaders who can champion this transformation and cultivate a workforce that embraces innovation within the evolving landscape of pharmaceutical manufacturing.
About The Author
Erika L. Roberts, MFS, is principal consultant and owner of ELR Lab Services LLC. Having more than 15 years of experience working in many different areas of the pharmaceutical/biotech manufacturing quality environments, she has particular expertise in sterility testing, microbial identification training, HPLC analysis, cGMP training, analytical chemistry, and pharmaceutical regulations. Roberts obtained a master’s in forensic science in 2006 with an emphasis in document examination.