Small Molecule Drug Discovery: Can AI Do It All?
By Anirban Datta, Ph.D., Verseon International Corporation
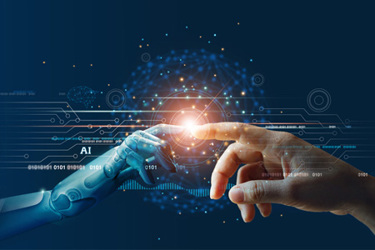
In 2020, a Tesla owner posted a funny video of his car mistaking a Burger King sign for a stop sign. This incident was a harmless example of a type of advanced machine learning algorithm called deep learning making an error. Although Tesla Motors is well known for applying artificial intelligence (AI) in their cars, it turns out that these AI algorithms are far from perfect.1 Sadly, there are far more dangerous examples of AI-based self-driving software making serious mistakes that could lead to injury. One such example involved another Tesla vehicle that could not recognize a person in the middle of the intersection holding up a stop sign. The onboard AI decided not to stop the car.2 Thankfully, the human driver intervened to prevent tragedy.
Despite some glaring shortcomings, deep learning has garnered much attention in recent years because of its utility in various real-world tasks that used to require human intervention. Examples include image and speech recognition, handwriting analysis, and so on. The recent successes of deep learning have given rise to optimism that it can now solve much harder problems, including the discovery of new drugs to treat human diseases.
Modern drug discovery struggles with enormous cost and inefficiency. Currently, a typical drug takes 10 to 12 years to develop and costs billions of dollars prior to approval, not to mention that most drugs fail in clinical trials. Undoubtedly, there is room for improvement. The hope is that AI can solve the single most challenging problem at the heart of modern drug discovery: the reliable prediction of novel small molecule drugs that potently bind to a disease-causing protein and alter its function.
Finding Novel Drugs Efficiently Remains The Biggest Challenge
Over the last decade, many companies have been founded with the promise of using AI to revolutionize small molecule drug discovery. Both private and public market investors have been pouring money into these companies. Exscientia and Recursion Pharmaceuticals currently lead the pack in terms of fundraising, while others like InSilico Medicine, InSitro, XTalPi, Generate Biomedicines, Benevolent AI, and Atomwise are not far behind. Despite the numerous AI drug-discovery companies now crowding the space, investor interest has shown no signs of abating, and new companies are still being formed and funded.
But will any of these companies succeed?
In this context, we define “success” as the use of AI to reliably predict novel small molecule drugs that can be brought to the market and would otherwise have been highly unlikely to discover. But if hype about applying prior new technologies in drug discovery is any indication, it is unlikely that any of these companies will realize the full measure of “success.” While we don’t have a crystal ball, we wondered if it is possible to predict, based on past and future trends in machine learning and the unique challenges of rational drug design, what kind of company is most likely to succeed — whether on the list above or not.
Success Of AI Depends On The Availability Of Copious Relevant Training Data
First, it is worth taking a quick look at what really powers machine learning. The success of machine learning, and in particular deep learning, depends heavily on the availability — and quality — of large data sets for training. Data, in particular dense data sets that include all possible relevant scenarios, allow an AI model to make inferences based on what it “learns” in training. In general, the more training data there is available, the better most AI models perform.
In addition, typical AI models are essentially black boxes, and predictions made by these models are practically impenetrable to a human. Relying on these predictions then requires trust in the AI model, which is further complicated by the fact that in most applications it is not possible to train the AI algorithm on all possible scenarios. Humans are intuitively good at making logical leaps. AI models, as it turns out, are not — at least not yet. A real-world example of this is that car encountering a crossing guard holding a stop sign and failing to stop. Unlike the AI that was unable to make a correct decision when confronted with a situation outside its training data set, the human driver was able to extrapolate and made the right choice.
Training an AI model to predict novel small molecule drugs also requires an enormous amount of data because of the complexity involved in protein-small molecule binding and the sheer number of possible small molecule binders. The problem is that there are many orders of magnitude more potential small molecule drugs that can bind to disease-causing proteins than are represented in available data. All the experimental data from all small molecule drug discovery programs in the world amount to a small collection of tiny tide pools on the edge of a vast, unexplored ocean of possibilities for which no binding data exists. This dearth of training data is a big problem for effectively training an AI model. AI is good at interpolating features within the bounds of a well-explored pool of training data but unable to make useful extrapolations far outside it.
Deep Learning And Protein Folding
But didn’t DeepMind’s AlphaFold 2 just take a major leap forward in protein structure prediction using AI? Indeed, it did. However, a quick look at how this breakthrough occurred will also shed some light on why the challenges facing AI-powered drug discovery won’t be as easy.
For proteins, there are several large genomics databases containing vast numbers of protein sequences across many species. Proteins share more structural (and functional) similarities to other related proteins than one would surmise based on protein sequence similarity alone, even when comparing across species. Searching these databases yields homologous proteins for a given query protein sequence. One can then align the query protein with these homologs to build a multiple sequence alignment (MSA). Lining up related protein sequences as rows of an MSA causes useful patterns to emerge. For example, when an amino acid in a given position changes, another one some distance away also changes. These pairwise correlations form the basis for a well-known biological principle called co-evolution. They also indicate the likelihood that the two amino acids contribute to the protein’s structure and will be in proximity in the final 3-D folded shape, irrespective of how far apart they are in the protein sequence. And if some of the homologous proteins in an MSA also have empirically determined structure, then so much the better. Even distant homologs with low sequence similarity can be structural templates to predict folding. This technique is known as homology-based modeling.
Like other AI predecessors applied to protein folding, AlphaFold 2 seeks to maximally exploit the information content in large genomics databases using MSAs, co-evolution, and structural templates. While DeepMind implemented several AI innovations in AlphaFold 2 in its quest to crack the grand challenge of protein folding, the rapidly increasing availability of high-quality training data in recent years — both in terms of protein sequences and experimentally determined protein structures — played a central role.3 Indeed, AlphaFold 2 was trained on immense data sets from publicly available genomics databases with hundreds of millions of protein sequences4,5 and databases containing almost 175,000 protein structures,6 in order to build MSAs and find structural templates. The three bioinformatics pillars, MSAs, co-evolution, and homology modeling, empowered AlphaFold 2’s AI with critical training data without which its breakthrough in protein structure prediction would not have been possible.
Predicting Protein-Small Molecule Binding Is A Vastly Different And Much Bigger Challenge
Protein-small molecule binding is a harder problem to solve using AI than protein folding. There are a variety of reasons it is more difficult, including the lack of apparent analogs for MSAs, co-evolution, and homology modeling for protein-small molecule binding. But ultimately there are three main reasons why the sparseness of available training data hurts AI-based drug discovery.
First, an AI applied to protein-small molecule binding will be biased toward predicting drugs similar to those on which it has already been trained, because it can interpolate but not extrapolate from known data. The sparsity of experimental binding data therefore restricts the type, number, and variety of drug-like molecules AI can find. At best, AI offers an incremental improvement on known molecules. But it is ill-suited to discover drugs that do not resemble known compounds. The situation gets even worse when focusing on new protein targets that do not have a well-characterized set of known binders.
Second, for efficient training, AI should have access not only to positive binding data but also to negative data. In other words, the AI needs to learn from both what binds and what doesn’t bind or binds weakly, so that it can make reliable predictions. Negative information is even harder to come by, since most research publications and patents will only describe compounds that have positive results.
Third, protein-small molecule binding is acutely sensitive to slight changes. Seemingly minor changes to the chemical structure or 3-D coordinates of a small molecule can lead to significant differences in binding affinity. Such abrupt changes are difficult for deep learning to accurately predict without staggering amounts of dense and relevant data.
The Path Forward For AI-driven Drug Discovery
How do we pick the (likely) winners in the race to discover novel drugs using AI?
Because the availability of sufficient training data is a serious limitation, the big pharmaceutical companies with their large and proprietary drug discovery data sets accumulated over many decades may seem to have an advantage. AstraZeneca, Merck KGaA, Novartis, and GlaxoSmithKline have all started their own in-house AI-enabled drug discovery efforts. While Big Pharma companies can leverage their own historical data, most of the data is legacy information from past drug discovery campaigns and are not diverse or dense enough to effectively train an AI. Nor are these data sets relevant to entirely novel chemical entities.
The question then becomes how to get around this experimental data bottleneck.
The answer may lie in advanced physics-based molecular modeling. Physics-based molecular modeling uses the fundamental principles of molecular interactions to predict the binding strength of a protein and a small molecule. This technique generates synthetic data and replaces expensive, time-consuming experiments. As the synthetic data build clusters, AI can then interpolate to find novel drug-like binders that do not resemble the current pharmacopeia.
While AI is good at interpolation when trained on large data sets, molecular modeling is well equipped to extrapolate based on the rules of molecular physics. Properly integrating AI and molecular modeling is likely to produce far more powerful breakthroughs in small molecule drug discovery than either approach could individually. Hence, companies with deep expertise in both physics-based molecular modeling and AI may have the ultimate advantage.
References
- Rapier, Graham (2020, June 25) Tesla's Autopilot confused a Burger King sign for a stop sign. Business Insider. https://www.businessinsider.com/tesla-autopilot-mistakes-burger-king-stop-sign-new-ad-2020-6
- Marcus, Gary (2022, March 10) Deep Learning Is Hitting a Wall. Nautilus. https://nautil.us/deep-learning-is-hitting-a-wall-14467/
- Jumper J, Evans R, Pritzel A, Green T, Figurnov M, Ronneberger O, Tunyasuvunakool K, Bates R, Žídek A, Potapenko A, Bridgland A, Meyer C, Kohl SAA, Ballard AJ, Cowie A, Romera-Paredes B, Nikolov S, Jain R, Adler J, Back T, Petersen S, Reiman D, Clancy E, Zielinski M, Steinegger M, Pacholska M, Berghammer T, Bodenstein S, Silver D, Vinyals O, Senior AW, Kavukcuoglu K, Kohli P, Hassabis D. Highly accurate protein structure prediction with AlphaFold. Nature. 2021 Aug;596(7873):583-589. PMID: 34265844
- UniProt Consortium. UniProt: the universal protein knowledgebase in 2021. Nucleic Acids Res. 2021 Jan 8;49(D1):D480-D489. PMID: 33237286
- Mitchell AL, Almeida A, Beracochea M, Boland M, Burgin J, Cochrane G, Crusoe MR, Kale V, Potter SC, Richardson LJ, Sakharova E, Scheremetjew M, Korobeynikov A, Shlemov A, Kunyavskaya O, Lapidus A, Finn RD. MGnify: the microbiome analysis resource in 2020. Nucleic Acids Res. 2020 Jan 8;48(D1):D570-D578. PMID: 31696235
- Velankar S, Burley SK, Kurisu G, Hoch JC, Markley JL. The Protein Data Bank Archive. Methods Mol Biol. 2021;2305:3-21. PMID: 33950382
About The Author:
Anirban Datta, Ph.D., is the head of discovery biology at Verseon International Corporation. He has over 20 years’ experience in biomedical research and pharmaceutical drug discovery. He is the driving force behind Verseon's automated processes for biological characterization of compounds, teasing out their unique properties, and structuring drug candidate development pathways. He has led multiple drug discovery programs in diverse disease areas, including cardiometabolic disorders, ophthalmology, and oncology. Datta was previously a scientist and Susan B. Komen Breast Cancer Foundation Fellow at UCSF and the recipient of lung and breast cancer concept awards from the U.S. Department of Defense. His early research was spun out into a cancer diagnostics company. He received his B.S. in physics and biology from the University of Chicago and his Ph.D. in molecular biology from the University of Pennsylvania.