New Neuroscience-Based Human Factors Research Methods Will Improve Devices' HFE Performance, Simplify FDA Approval
By Charles L. Mauro, Mauro Usability Science
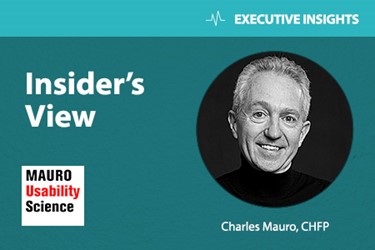
This is the first of a two-part series taking a hard look at current human factors engineering (HFE) testing methods and looking at new advanced testing methods that HFE teams can utilize to improve the HFE performance of medical devices. Part two of this article will be published June 14, 2018.
Human factors engineering and related usability testing is a critical aspect of FDA medical device guidance that has led to improvements in HFE performance for many medical devices. However, the methodologies employed by those conducting HFE optimization studies and usability testing — as discussed in both HE75 and the FDA’s HFE guidance — are based on surprisingly out-dated research methods, developed more than 100 years ago by Fredrick Taylor and others.
Current HFE research employs simple task-based observation of patient behaviors, “think-aloud” descriptive processes, and debrief interviews. The last major advance in traditional HFE testing was the addition of video recording for post-study review introduced 30 years ago. Even though these traditional methods are well understood to be highly subjective, error prone, and subject to all manner of self-reporting biases, they continue to be the methods of choice for the vast majority of HFE device usability studies. Even current FDA guidance and HE75 proffer such methods as the primary means of assessing devices’ HFE performance.
It is important to note that these traditional observational methods have value when assessing HFE performance, but there is now a pressing need to supplement subjective, observation-based testing methods with more objective, neuroscience-based data capture systems. The combination of testing methods will produce much more usable and productive medical devices, as well as improved clinical outcomes and reduce FDA review and approval processes.
On a higher level, HFE testing as a professional discipline has not taken advantage of a wide range of advanced and well-understood physiological, biomechanical, cognitive, and emotional sensing and testing modalities. For more than a decade, these new systems have provided medical research professionals and associated engineering teams with a vast and powerful new set of data focused on understanding human behaviour in a wide range of domains.
Application areas for these new testing systems include neuro-marketing, cognitive neuroscience research, applied medical research, aerospace, aviation, transportation, athletics, education, consumer products and a wide range of military applications. These commercial domains have embraced advanced neuroscience-based testing based on an understanding that better overall system performance demanded much deeper understanding of human response to new technology.
New Methods For New Device Design Problems
Traditional HFE testing methods may have been appropriate for simple, traditional medical devices, but the incoming generation of devices presents to patients and HCPs more status indications, more complex errors states, more complex interaction behaviours, less familiar device form factors, new IFU formats, more complex drug delivery schedules, and new, larger-molecule biologics, as well as a new generation of wearable devices and associated smartphone apps.
The time has arrived to supplement traditional observational methods with scientifically-valid HFE testing methodologies that support the creation of medical devices (and related medical technology) that are objectively focused on meeting the cognitive, emotional, psychological, and physiological needs and limitations of patient populations who differ from the healthy population in important ways that measurably impact clinical outcomes.
Neuroscience-based data-capture systems can produce unified data streams that provide device engineering teams with unprecedented access to valid HFE research data, which can be utilized to objectively optimize medical device HFE performance, as well as all manner of IFU and package design solutions.
This approach also will aid the FDA in defining new HFE performance requirements and related testing methods, as well as shorten the time required to conduct HFE reviews and certify compliance. It is only a matter of time before the FDA begins moving toward neuroscience-based HFE performance guidance that is based on objective and engineering-focused HFE criteria derived from the application of advanced HFE research methods. Other major governmental agencies have defined product performance guidance in science-based terms for decades. Further, the opportunity to base patent claims on objective HFE performance improvements is a major benefit for those creating new medical device solutions.
New sensing modalities now make it possible to capture data on a wide range of physical device states — including device location, angle of injection, depth of injection, interaction 3D profile, patient hand tremor levels, and interaction forces — previously impossible to measure accurately and at reasonable cost. Neuroscience-based data capture also can measure cognitive and emotional responses to medical devices, including cognitive workload, emotional response, and learning decay.
These new methods can be applied to simple, single action auto-injectors or highly complex augmented reality robotic surgical systems. Finally, in addition to creating new devices, these methods can be used to optimize existing devices’ hardware, software, and apps.
New Device And Patient HFE Testing Modalities And Technologies
- 3D Spatial Tracking — A high-precision sensor system created to record micro-levels of object motion as users interact with a product or other physical object. It provides a highly refined view of the impact of design shape on a user’s ability to manipulate a device or product in a 3D space. It also imparts a vast data set that is used to optimize shape of products, package designs, or other objects. It also is used to measure the impact of design on a patient’s ability to self-administer drugs using new drug delivery devices or syringes.
- Newtonian Force Measurement — Measured by a network of force sensors and related software and hardware that can interface with any medical device solution or related component of a larger design. It allows for a highly accurate measurement of forces required on the part of the user to manipulate a solution’s overall design, individual component parts, or design concept during use. This method also can be utilized to generate formal user needs data and related functional limitations, in terms of force exertion and manipulation required by a given medical device.
- High Definition Electromyography — Provides a high-resolution view of the physiological effort involved in manipulation of medical device design solutions or concepts. It records the activity of core muscle groups involved in manipulation of a design solution, as well as yields the direct physiological measure of user effort, which often is highly correlated with user preferences.
- Galvanic Skin Response — A data stream utilized to measure the intensity of users’ emotional responses to device designs, device complexity, IFU comprehension and device design concepts as they interact with an actual device, simulated device, or content related to a medical device design solution. It contributes insights that users frequently cannot articulate using traditional interview methods. Used in combination with other methodologies.
- Electroencephalogram (EEG) — Widely promoted as a means of assessing consumer response to all manner of device design solutions, this system offers insight into users’ higher-level cognitive processes as they interact with device design solutions or view solutions in various media. Can produce critical insights into cognitive workload associated with use of a medical device and related IFU content. Can be utilized in conjunction with eye-tracking data.
- High-Resolution Eye Tracking — A data stream that provides insights into how the user attends to the visual aspects of a device design solution, in real time. Combined with other forms of data capture, it imparts robust insights into the visual attention differences between design concepts that impact user behavior and acceptance. Can be utilized in lab-based settings, as well as “in the wild” data capture, in which case the process utilizes specialized data-capture glasses. Eye-tracking is common in traditional studies, but application of ET findings into a larger data stream has produced a new way to utilize those insights.
- Facial Expression Analysis (Emotional Response) — An artificial intelligence-based system for capturing the expressed emotional responses of users viewing or interacting with device design solutions. This can include medical device products, patient treatment environments, printed material, and video content. It allows for deep and compelling insights into which features and/or combinations of features in a design solution elicit expressed facial emotions.
- Automated Task / Usability Heuristics — A data capture and professsional audit system and related observational tool that yields highly detailed data on the time, errors, and confusion present when a user interacts with a given device design solution. The system is fashioned to produce usability testing insights for use in design optimization, and can be utilized to identify performance problems captured in prior HFE studies that are known to produce errors and confusion, thus allowing for removal of such design features during early design development. System is based on creation of a database of device design HFE performance ratings correlated with device interface design features.
- Learning Transfer Analysis — A data capture system designed to determine whether changes to a design or related interface are going to have major negative impact on previous users of the system. Combined with other data streams, it reveals which design changes will have negative, neutral, or positive impact on a given design solution. This system utilizes various data streams to address FDA guidance related to Threshold analysis.
- Cognitive Workload Analysis — Software-based data capture systems that allow for the calculation of the cognitive workload associated with understanding and using medical devices by specific patient populations. It provides objective measurement of how much cognitive effort design solutions require on the part of users during various, critical interaction sequences, or simply when viewing the design solution without interaction.
- Information Foraging Theory / Behavior Modeling — A data capture and behavior modeling system that allows for the creation of software-based predictive models of user behavior, based on input from other data capture systems discussed above. It provides a rapid means of assessing the relative impact that design solutions will have on users’ ability to locate, integrate, and take action on critical content, features, or functions without creating complex physical models, simulations, or test setups.
- Interactive Online Surveys and Subjective Response System — An extensive array of online data capture systems based on professionally audited and tested survey tools that integrate seamlessly into the data capture streams discussed above. The system utilizes a combination of industry-standard and proprietary question sets, ranking systems, and scales to capture subjective response to design solutions in a range of research settings. The system can be utilized to support design concept testing, including the implementation of advanced methods, such as MDS and CJA
- Data Stream Integration System — The data capture modalities discussed above individually produce massive amounts of real-time data. When the data streams are combined, they impart truly staggering amounts of data, which require specialized data integration and data analysis tools. Working with industry-leading data integration supported by development of proprietary software integration systems, these new systems form a critical bridge between data and insights.
- Data Clipping, Scrubbing, And Data Analysis System — Due to the complex nature of the data integration streams required to execute robust HFE evaluations, it is necessary to utilize advanced data clipping, scrubbing, and statistical analysis routines. To solve this problem, there are proprietary data capture and analysis tools that allow for effective execution of the complex statistical analysis required to provide clients with reliable design research suitable for making major corporate investments in specific design solutions or directions. The data stream aggregation tool interfaces with audited and industry-standard advanced statistical software systems.
These new data capture methods already have been successfully integrated and utilized to produce powerful insights related to medical devices’ HFE performance, instructions for use, and packaging. However, it is important to note that these new systems do not totally replace traditional observational research; they provide a large measure of objective data that verifies, builds upon, and augments our understanding of medical device HFE performance.
However, creating advanced HFE testing systems is not without its challenges. Part two of this series, slated for publication on June 14, 2018, will discuss the challenges and benefits of employing new advanced neuroscience-based testing methods in the creation of improved medical devices and related procedures.
About The Author
Charles L. Mauro, CHFP is Founder and President of MAURO Usability Science, a New York-based consulting firm founded in 1975 specializing in advanced human factors engineering research and the optimization for complex medical devices and related technology. He is Chairman of the Design Protection Section for the Industrial Designers Society of America and is the IDSA liaison to the US Patent and Trademark Office. He has lectured on product design and human factors engineering research at MIT, Stanford University, UPenn, and many other leading human factors research and engineering programs. Over his 47-year career Mr. Mauro has managed over 4,000 HFE research and development projects.
Mr. Mauro holds a BS with distinction in Industrial Design from The Los Angeles Art Center College of Design and a Master’s Degree in Ergonomics and Biomechanics from New York University. At NYU he was an appointed NIOSH Research Fellow at the RUSK Institute of Rehabilitation Medicine. His experience with complex medical technology spans more 47 years.
Reviewers: The author would like to thank the following reviewers. The content of this article is based solely on the opinions of MAURO Usability Science and, specifically, the author. It may not reflect opinions of the reviewers or their organizations.
- Conne Bazley, Ph.D., CHFP, Principal Human Factors Engineer, Pfizer, Inc.
- Brendan Murray, VP Enablement Services, iMotions, Inc.
- Elexa St. John-Saaltink, PhD, Research Scientist, MAURO Usability Science
- Chris Morley, MS Senior Human Factors Engineer, MAURO Usability Science
- Hannah Kang, MS Human Factors Engineer, MAURO Usability Science