Embracing AI-Driven Technology In Drug Development, Manufacturing
By Jana Spes and Ajay Pazhayattil
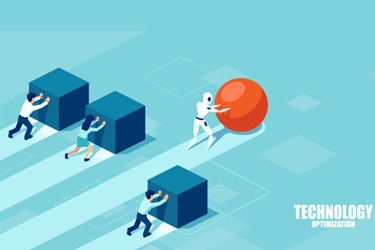
The drug development journey, which spans more than a decade,1 has encountered a persistent challenge: when products reach the market, the technology employed in their early stages becomes outdated and financially burdensome. However, with its transformative potential, artificial intelligence (AI) has the potential to bridge the gap. In this article, we delve into how AI can revolutionize accelerated pharmaceutical manufacturing technology adoption and market access for new molecules. AI already delivers value in drug discovery in various ways, such as access to new biology, improved success rates, and improved market prediction and analysis.2,3 The AI-driven drug discovery industry has grown significantly over the past decade, fueled by new entrants in the market, significant capital investment, and technology maturation.4 Generative AI rooted in large language models like ChatGPT has been a hot topic recently, with its potential for groundbreaking advancements across scientific disciplines, particularly medicine.5 Furthermore, an exciting investment landscape is emerging, spurred by the rise of generative AI, with venture capital firms fervently backing startups focused on developing AI-based applications.6
Pharmaceutical technical development and manufacturing can benefit from using large language model (LLM) generative AI systems in several ways. During the 12 to 15 years it takes to develop a product, enormous amounts of data are generated and processed, problems are continuously solved, technical questions are answered, decisions are made using science, similar product/process data is utilized,7 and new knowledge is generated. An AI application can use all available knowledge, literature, and prior art, incorporate newly generated data and knowledge management, and provide new insights. The models can learn along the way. An LLM is a generative AI that processes vast amounts of text and generates new text based on the patterns it identifies.8 It is ideal for assisting development scientists in doing development work more efficiently, faster, and smarter.
Embracing Enhanced Technological Platforms
In pharmaceutical manufacturing, migrating to improved technology platforms offers significant opportunities for advancements in patient safety and product reliability. Specifically, in the areas of drug-device combination products, cell and gene therapy (CGT) products, and in continuous manufacturing technology, AI can emerge as a key catalyst. The crux of this opportunity lies in leveraging AI to integrate the latest pharmaceutical technologies directly into product manufacturing at the time of launch rather than relying on outdated technologies employed during early-stage development. In the pursuit of adopting improved technology platforms in pharmaceutical manufacturing, patient safety must remain paramount. It is crucial to utilize flexible regulatory tools, such as ICH Q12, which can enable enhancements to be implemented before the product launch.9 A quality by design (QbD) process development undergoes meticulous process characterization along with a deep understanding of the impact of each process parameter and attribute. Therefore, any technology that can later demonstrate the ability to reduce variability further and consistently deliver high-quality products should be embraced and justified with data gathered through LLMs.
LLM Applications
In small molecule development, simplifying Phase 2 formulation and manufacturing processes can yield significant benefits. By reducing excipients and processing steps, sponsors can achieve the same level of quality and efficacy while improving processing efficiency and reducing the cost of goods sold.10 Multiple factors influence the quality, safety, and efficacy of the final drug product, such as the choice of excipients, processing steps, and analytical methods. Leveraging extensive analytical and stability data, LLMs can confirm the absence of negative impacts on the quality profile. A shift toward residence time distribution (RTD)-based continuous manufacturing instead of a series of discrete unit operations presents an opportunity to enhance efficiency and minimize variability significantly. AI can be crucial in material characterization modelling, such as powder rheology, enabling precise control and optimization of manufacturing parameters.11 AI can now help design multistep organic syntheses facilitated with state-of-the-art robotics and reconfigurable reaction platforms such as flow chemistry.12
During the transition from proof of concept to pivotal clinical studies, the exploration of new cell lines and purification processes becomes essential. Sponsors are driven by a desire for improved efficiency and efficacy. In this scenario, leveraging AI can be beneficial. Sponsors can conduct a comprehensive evaluation of all available data to arrive at the optimal cell lines and purification technology using AI-guided analysis. This enables informed decision-making, facilitating the selection of the most suitable cell line and purification process. AI can also classify cell lines with similar morphologies and identify cross-contaminated cells, as demonstrated in a study that used a novel AI platform with three deep learning networks.13 Live-cell imaging and analysis, which combines microscopy techniques and powerful analysis software, can also be used to derive meaningful data with sensitive, real-time live-cell measurements.14
Efficiency gains can be achieved in the pharmaceutical industry, regardless of whether products are developed in-house, through hybrid approaches, or in partnership with a CDMO. Starting from the inception of each new molecular entity, various test methods, product prototypes, in-process data, release analytical data, knowledge, and processes are generated. It is crucial to gather, develop, document, and summarize all these essential elements to strengthen regulatory submissions. The use of LLM-based AI in pharmaceutical process development can help to improve the efficiency and quality of the manufacturing process, reducing costs and improving patient outcomes.15 Integrating AI and ML in QbD process development can help provide real-time visibility of how changes causing variability/bias impact product quality.16 The models can leverage massive amounts of data into improved process understanding and move toward a prescriptive, innovative pharmaceutical development process. Several AI solutions can adapt to new data sets or learn automatically and create new causal links between process parameters and process variables.17
The collection, management, and utilization of specific process data drive the AI revolution in pharmaceutical manufacturing. These data sets will be the basis for advancing our use of AI. Additional opportunities exist in automating electronic Common Technical Document (eCTD) module development data validation, regulatory submission, and lifecycle management. Applications that extract relevant data, autogenerate regulatory documents, track compliance, and improve regulatory outcomes by using data-driven insights for proactive regulatory management are already being built.18
Summary
The successful integration of AI into pharmaceutical development and manufacturing necessitates close collaboration with regulatory agencies and a commitment to continuous improvement. AI systems based on LLMs have demonstrated immense potential, while the FDA has emerged as an active partner in AI implementation, as communicated to the industry.19 The agency’s recent call for AI implementation in development and manufacturing20,21 underscores its commitment to fostering innovation and facilitating meaningful conversations around adoption. The FDA notes the potential for AI/ML, in concert with other advanced manufacturing technologies, to enhance process controls, increase equipment reliability, monitor early warnings, detect recurring problems, and prevent batch losses.
Over the next decade, significant technological advancements are expected to occur before products undergo Phase 3 studies. In Phase 1 and 2 trials, the number of patients involved is limited, and the risks of adverse effects are relatively low. However, the development of products often spans several years before they can be used in a larger population for Phase 3 studies. Upgrading various aspects of drug product technology to meet current standards and utilizing AI-enabled comparability assessments to bridge the gap with the original formulation will bring a range of patient benefits, including reduced variability, risks, and costs.
References
- https://www.ncbi.nlm.nih.gov/books/NBK22930/
- https://www.ncbi.nlm.nih.gov/pmc/articles/PMC7577280/
- https://www.bcg.com/publications/2022/adopting-ai-in-pharmaceutical-discovery
- https://www.mckinsey.com/industries/life-sciences/our-insights/ai-in-biopharma-research-a-time-to-focus-and-scale
- https://one.oecd.org/document/DSTI/CDEP(2022)14/FINAL/en/pdf
- https://www.deep-pharma.tech/ai-in-dd-q3-2022-subscribe
- https://www.fda.gov/files/drugs/published/Process-Validation—General-Principles-and-Practices.pdf
- https://legal.thomsonreuters.com/blog/from-hype-to-reality-what-businesses-need-to-know-about-generative-ai/
- https://database.ich.org/sites/default/files/Q12_Guideline_Step4_2019_1119.pdf
- https://www.ncbi.nlm.nih.gov/pmc/articles/PMC4590717/
- https://www.fda.gov/media/168879/download
- https://par.nsf.gov/servlets/purl/10190515
- https://www.ncbi.nlm.nih.gov/pmc/articles/PMC7327366/
- https://www.sartorius.com/en/products/live-cell-imaging-analysis/live-cell-analysis-software/incucyte-ai-cell-health-analysis-software-module
- https://www.proclinical.com/blogs/2023-4/top-20-artificial-intelligence-life-sciences
- https://pubmed.ncbi.nlm.nih.gov/26265189/
- https://nexocode.com/blog/posts/process-analytical-technology-pat-with-ai-in-biopharmaceutical-manufacturing/
- https://www.enkrisi.com/pharmwrite
- https://www.fda.gov/media/165743/download
- https://www.fda.gov/media/167973/download
- https://www.federalregister.gov/documents/2023/03/01/2023-04206/discussion-paper-artificial-intelligence-in-drug-manufacturing-notice-request-for-information-and
About The Authors:
Jana Spes, M.Sc., MBA, is a technical operations professional with breadth and depth of experience in solving development challenges. In her 20+ years career working with global companies in the U.S., Europe, and Canada, she served in leadership roles in CMC development, process scale-up, technology transfer, validation, and manufacturing. Her thought leadership explores ways of working between innovators and CDMOs. Spes has published innovative methodologies for application of FDA and ICH guidelines in product development that aim to ensure manufacturing process robustness and is one of the inventors on a patent describing methodology to implement FDA validation guidance. You can connect with her on LinkedIn.
Ajay Babu Pazhayattil, DBA, is a management consultant helping clients to achieve their business goals while adhering to global regulatory compliance mandates. Previously, he was in leadership positions at a major CDMO and generic pharmaceutical organizations. He has contributed to key remediations and strategic organizational initiatives. Pazhayattil’s doctoral research, "Machine Learning and Artificial Intelligence Strategies for the Pharmaceutical Industry," quantitatively identified the factors influencing the initiation, convergence prospects, ROI, and the variables that can delay ML and AI in the pharmaceutical manufacturing industry. You can connect with him on LinkedIn.