Climbing The Blockchain Ladder To Optimize Clinical Supply Forecasting
A conversation with Alexandru Popa, digital products consultant
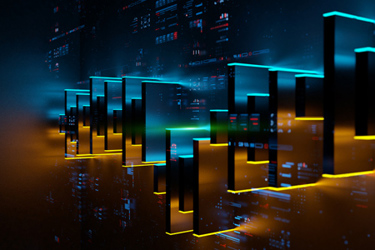
While most of pharma was going about its business, blockchain-based technology quietly infiltrated.
No, it did not become the blockbuster universal supply chain savior it was billed as 15 years ago. Instead, its applications are specific and supplemental.
More recently, Alexandru Popa, a digital products consultant who has been part of blockchain’s evolution in managing pharmaceutical supply chains, is focused on its potential to couple up with artificial intelligence and provide more reliable forecasts for clinical supply management.
We had some questions about how the technologies work hand in hand. Here’s what he told us.
How does blockchain technology help build robust AI-based clinical trial demand forecasting tools?
I see it as a logical progression. Blockchain lays the groundwork — it stores and structures the data, breaks down silos, and builds a common infrastructure. That’s one of the key challenges today in clinical trial supply chain data — fragmentation. So, if we start with blockchain, we’re essentially setting the stage for AI to build on top of it.
Blockchain is primarily used for track-and-trace purposes because of its immutable nature. Once a record is written to the blockchain, it can’t be altered. That immutability makes it a reliable source of truth.
This is especially valuable in ecosystems with multiple participants — like clinical trial supply chains, which involve manufacturers, distributors, wholesalers, pharmacy chains, clinics, and clinical sites. As goods move through the supply chain, blockchain helps track each step, providing visibility and trust.
Clinical trials also face unique challenges — unpredictable patient recruitment that varies by region and indication, high dropout rates, frequent protocol deviations, and large swings in demand. On top of that, lead times and product shelf life are major constraints — particularly for oncology drugs or vaccines with very short lifespans.
This is where AI adds value. By enhancing real-time data analysis, it can detect anomalies and send alerts when something doesn’t look right.
We can also break this down by use case — for instance, forecasting and resupply. AI enables inventory optimization and minimizes under- or overstocking. You can optimize depot sites, monitor quality more proactively, and flag bottlenecks in the supply chain by analyzing routing rules and lead times.
Another emerging benefit — though I haven’t experimented directly with this — is scenario simulation. With the right data, you can do scenario simulation with AI to support risk planning. This concept is also gaining traction in smart manufacturing.
What's the current state of adoption? The embrace of blockchain in general still appears limited.
Most pharma companies are still in the early to middle stages of adoption. There are various pilots and proof-of-concept projects, especially in large pharma, but we haven’t yet seen widespread full-scale implementation.
One of the main roadblocks is fragmented data. As I mentioned, blockchain can help solve that, but the broader issue is that companies are still experimenting. Few have moved beyond pilots. It’s a chicken-and-egg problem. Without standardized data, it’s hard to build systems that can talk to each other.
In a project I worked on, which was focused on the pharma supply chain, we achieved some success in live deployments in a few Asia Pacific markets. But when we tried to scale, we ran into challenges. For example, even when using a standard like EPCIS (electronic product code information services), which is supposed to be the language of supply chains, everyone still uses their own codes and terminology.
One company might use the term “shipped,” while another might call it “dispatched.” So, before you can truly break down silos, you have to agree on what each event means. And that’s not just a supply chain problem — it’s true for all types of clinical and operational data. Every system labels and formats its data differently.
There have been some notable initiatives. For example, Sanofi is using AI to simulate oncology clinical trials. But overall, we’re still dealing with low data interoperability, a lack of standardization, and a need for greater organizational readiness and data literacy.
There’s also the human element — resistance to change. Even when pilots show promising results, once you move to production, things can fall apart if people don’t follow through or resist new processes.
Is there uncertainty about how much automation is acceptable in GMP decision-making?
Yes, definitely. There’s a lot of uncertainty around that. When you're operating in a GMP environment, any decision made by AI must be validated, explainable, and compliant. Many companies are still trying to figure out how much automation is acceptable in these high-stakes scenarios.
Are there patient data or privacy risks?
That’s a tricky topic, and I don’t want to be misunderstood. Blockchain is secure — but the type of blockchain used in pharma today is typically private and permissioned. It acts more like a decentralized database. This is intentional. It's cheaper, faster, and trust isn’t as big an issue because the participants (e.g., clinical sites and distributors) already have established relationships, so you don’t have a big need for high security, high decentralization. The high decentralization of Layer 1 public blockchains is not a critical requirement for current blockchain use cases in pharma and is often traded off for greater control and flexibility.
We don’t use public blockchains like Ethereum or other highly secure, trustless networks. That said, the privacy risks are more relevant on the AI side.
AI can identify patterns in data — especially when combining data sets. For example, if you merge location, disease type, and ZIP code, the AI might be able to re-identify a patient. Add in electronic health records or imaging data, and the risk of re-identification increases significantly.
But for forecasting, you don’t necessarily need granular patient data, right?
That’s correct. You don’t need granular patient data to forecast. But at the point of ingestion — when the drug reaches the patient — additional data is captured.
Let’s say a specific pack leaves the warehouse for a randomized patient ID. Once the drug reaches the clinical site and is administered, doctors typically record additional patient details. From a supply chain perspective, this is when the product status shifts to “delivered” or “consumed.”
You can aggregate that data later, and yes, it can include patient identifiers. So even though the forecasting itself doesn’t require patient-level detail, that information does become relevant at the endpoint.
What are your projections for the future? Will the industry ever fully standardize and "speak the same language"?
There’s been real progress, but the main challenge remains: we need a common infrastructure for data. Right now, the industry is fragmented. Traditional planning models are too static — they don’t adapt well to real-world variability or uncertainty.
Regulatory complexity adds another layer of difficulty.
I believe meaningful adoption will start with decentralized clinical trials and personalized medicine. These areas are still relatively new and don’t have legacy systems or entrenched standards. It’s also very difficult to create one-size-fits-all standards for these models, which might actually make them more adaptable.
About The Expert:
Alexandru Popa is a digital transformation consultant specializing in leading global technology initiatives in the pharmaceutical industry. His work includes the development of blockchain-enabled track-and-trace systems, digital biomarkers, innovative mobile applications, and AI-powered data tools. He has held contract roles with leading pharmaceutical companies such as Merck, Roche, and Novartis.