Autonomous Decision-Making: Are We Ready for Agentic AI

By Louis Garguilo, Chief Editor, Outsourced Pharma
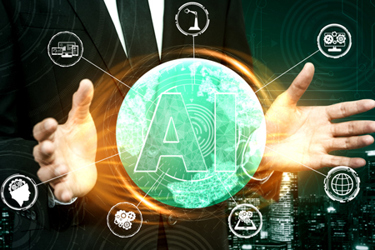
The biopharma industry has generative artificial intelligence (AI) in its employ. After a long wait, we’ve adopted it rather suddenly.
Just in time for a new kid in town. Next to ride in is Agentic AI.
This AI iteration will more robustly revolutionize our drug development and manufacturing, and impact, for example, outsourcing decision-making, and CDMO relationships.
Regarding “regular” AI – our generative, large language-based intelligence applications – if you work in drug discovery, it’s likely you’ve been utilizing this for some time. It helps you understand which molecules and materials – their shapes, expected toxicities, and other key attributes (such as cost) – are most suitable as development candidates.
I recently wrote about the process chemists and “route scouters” in development who now utilize data and artificial-intelligence infused tools.
Our processing equipment and manufacturing facilities have gotten smarter, arrayed with sensors and monitors, capturing data in real time, for example at a CDMO which then sends it on to sponsors.
But it appears we haven’t seen the half of it yet.
We’ll unpack the newly emerging – and somewhat frightening – technology in two editorials, starting here with an overview.
In part two, we’ll suggest ways in which Agentic AI could bring transformative efficiencies and capabilities to CDMOs to make them more integral in supporting biopharma organizations of all kinds.
Agentic AI, you’ll learn, may soon be making your decisions for you.
What’s The Difference?
In essence, generative AI is about creation. Agentic AI is about taking (autonomous) actions.
Functionally, then, generative AI produces novel outputs based on prompts.
It does not inherently have decision-making or goal-oriented capabilities on its own; its output (while extremely valuable) is pretty much static, and relies on human analysis and human’s taking actions based on such output.
It has also become quite effectively communicative, able to hold sophisticated "conversations" and discussions with humans.
Agentic AI is designed to analyzie data and inputs as well, and then autonomously make choices and take actions to achieve specific objectives.
Robots come to mind, yes, but it is also embedded in software and hardware, and for us specifically, processing equipment and throughout supply chains.
This involves – as far as I understand it – a form of reasoning, learning from interaction and adapting to new information, rendering those inputs into dynamic operation, with the potential for continuous interactivity and feedback loops.
What Might It Do For You?
According to marketing research at IT consulting firm Gartner, within 3 years 15% of daily business decisions of all kinds will be made via Agentic AI exhibiting fully autonomous decision-making capabilities.
As a scientist, engineer, project manager, or of course at any level of executive, given a set of constraints or environments, are you ready for independent AI-actuation to achieve your goals?
For one thing – perhaps the thing – this will require a level of trust in AI beyond any we have achieved to date.
That’s because Agentic AI presents a more penetrating non-human sensing and perceiving of our current (human-)proscribed environment to make autonomous decisions that influence those environments to achieve specific objectives.
We're talking about real influence.
Should we give it the authority to do so?
In other words, while today’s generative AI requires – we tell ourselves – decision-taking based on the interdictions of human analysis and actions, to a large degree this will not be the case with Agentic AI.
Personally, I neither subscribe to (or unsubscribe from) these expanding AI ideals. In fact, I am habitually the skeptic in the room.
But it’s time for the biopharma industry to peak around the corner. Like initial AI generations, this next spawning is sure to enter our labs, plants, meeting rooms and C-suites, and certainly arrive at our CDMOs, before we know it.
Humans Thinking About Thinking AI
For sure, humans haven’t been banished yet.
Allow me to quote NVIDIA’s Erik Pounds, director of product marketing, and other NVIDIA public statements. (For those unfamiliar, NVIDIA – more specifically its semiconductor products leading the advanced-AI revolution, is the most important AI enabler in the world today.)
Pounds has said Agentic AI is the next frontier of AI because it employs sophisticated reasoning and iterative planning to autonomously solve complex, multi-step problems.
That should enhance productivity and operations, and staying on focus, sounds like just what we’d like at our CDMOs.
Pounds has blogged that Agentic AI systems also ingest vast amounts of data from multiple sources, but does so to independently analyze challenges, develop strategies, and execute tasks like supply-chain optimization.
By the way, cybersecurity vulnerability analysis is among the other areas that will be positively impacted. It all sounds too good to be true.
How Does It Work?
Or perhaps I should ask, How does it think?
Quoting directly from NVIDIA, Agentic AI operates in a 4-Step Process aimed at problem solving:
- Perceive: AI agents gather and process data from various sources, such as sensors, databases and digital interfaces. This involves extracting meaningful features, recognizing objects or identifying relevant entities in the environment.
- Reason: A large language model acts as the orchestrator, or reasoning engine, that understands tasks, generates solutions and coordinates specialized models for specific functions like content creation, vision processing or recommendation systems. This step uses techniques like retrieval-augmented generation (RAG) to access proprietary data sources and deliver accurate, relevant outputs.
- Act: By integrating with external tools and software via application programming interfaces, Agentic AI can quickly execute tasks based on the plans it has formulated. Guardrails can be built into AI agents to help ensure they execute tasks correctly. For example, a customer service AI agent may be able to process claims up to a certain amount, while claims above the amount would have to be approved by a human.
- Learn: Agentic AI continuously improves through a feedback loop, or
“data flywheel,” where the data generated from its interactions is fed into the system to enhance models. This ability to adapt and become more effective over time offers businesses a powerful tool for driving better decision-making and operational efficiency.
Potential applications of Agentic AI are vast, and Pounds says limited only by “creativity and expertise.” (I think he means us.)
But again, in our industry, I’d add adoption will be limited by trust – that we have the safest solutions for patients – and by our regulatory bodies who help us make such determinations.
-----------
Next: A specific look at foreseen Agentic AI influences on outsourcing drug developmpent and manufacturing, and working with external partners.