AI Performance Management In Biologic And Drug Development
By Fahimeh Mirakhori
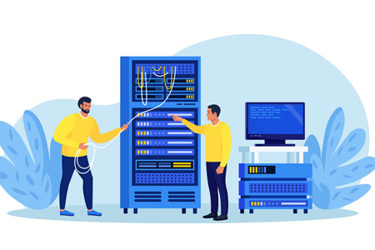
The evolving nature of AI requires constant life cycle management to ensure that AI models remain robust and that their performance is aligned with regulatory standards throughout their context of use (COU).
The concept of the “AI life cycle,” an essential part of the “Total Drug Product Life Cycle,” goes beyond the initial development and deployment of the AI model. It includes continuous re-evaluation and validations through a modular approach to ensure the AI model performance remains reliable as the model progresses through its COU life cycle. The FDA’s new draft guideline on AI1 and NIST AI Risk Management Framework (RMF)2 both emphasize similar considerations to ensure model performance integrity, which is vital for the effective application of core principles guiding AI maturity.1,2 These principles include transparency, dataset quality and integrity, explainability, accuracy, trustworthiness, bias control (algorithmic and non-algorithmic), safety, risk metrics, privacy, ethics, fairness, and governance, among other attributes.
Depending on the COU, each of these principles requires separate, clear, stepwise validation guidelines for determining the necessary level of human oversight. Initially, the AI system might operate with less autonomy and more human control, reducing technical complexity but increasing operational burden. The NIST RMF, which includes 90 tasks, focuses heavily on governance principles, with 38 tasks related to accountability, covering more than half of these principles. This alignment makes the RMF a robust tool for responsible AI management, akin to the FDA’s 7-step framework. Both frameworks emphasize continuous oversight for model integrity and accountability throughout the AI life cycle, with the FDA’s framework ensuring model credibility in regulatory decisions and the RMF providing long-term risk mitigation.1,2
The FDA and the EMA are increasingly managing diverse data inputs, ranging from raw clinical reports to real-world data and evidence (RWD and RWE) and electronic health records (EHRs).3,4 To ensure that AI models generate reliable and trustworthy outputs, it is essential that these datasets are of high quality, representative, and free from bias. AI systems must be designed and maintained with transparency in mind, as regulatory agencies need to interpret and justify the decisions made by these models. This guidance stresses the importance of AI models that can explain their outputs clearly, especially in high-stakes areas like drug development and patient safety monitoring. This transparency includes clarifying how data from clinical trials, RWD, and even nontraditional data sources are integrated into the AI model for regulatory decision-making. They also emphasize the necessity of continuous monitoring of AI models. This is especially crucial for high-risk applications where model drift can influence the safety and efficacy of the system. By continuously recalibrating AI models with new data, particularly data from RWE sources, the model can adapt to real-world changes while maintaining regulatory compliance. This life cycle management approach ensures that the FDA can evaluate AI models even after their initial approval, helping to maintain alignment with safety and performance standards as new data becomes available.
The concept of AI model life cycle maintenance is key to addressing the challenges in AI-driven drug development. The regulators recommend continuous updates to AI models to monitor and adjust for evolving data patterns, ensuring the models’ reliability and effectiveness over time. This ongoing maintenance is crucial, particularly in manufacturing, where AI applications must adapt to changes in production environments and regulatory requirements.
Life cycle management of AI models in drug development extends beyond the initial stages of model creation and validation. It demands multiple levels of modular controls and validations, with an initial focus on organizational resource management followed by more technical requirements as the model matures. Aligning this life cycle management with the FDA’s 7-step credibility framework ensures that AI models undergo rigorous scrutiny throughout their life cycle — from development through post-market surveillance. This framework, with its emphasis on defining the model’s purpose, measuring risk, and ensuring ongoing assessment, supports the maintenance of robust AI systems through continuous validation activities such as performance monitoring, data integrity checks, and transparency mechanisms. The International Society for Pharmaceutical Engineering (ISPE) AI maturity model guides the required validation levels by control design and autonomy.5 This model can be used as a strategic tool, dynamically guiding the AI system through its life cycle by balancing autonomy and control as necessary. By combining the FDA and ISPE frameworks, sponsors can ensure that AI systems evolve responsibly, continuously meeting regulatory expectations and safeguarding patient safety and product quality. This integrated approach is crucial for mitigating evolving risks, such as data drift and algorithmic bias, ensuring that AI-driven decisions remain accurate, reliable, and transparent throughout the drug product life cycle for the COU to ensure patient safety (Figure 1).
Figure 1. Mitigate the AI model performance integrity throughout the product development life cycle. Incorporating AI control design and autonomy levels with the FDA’s 7-step framework provides a structured life cycle management approach, ensuring continuous AI model validation. This process enhances AI model performance integrity throughout the drug development life cycle, from initial design to post-market surveillance and eventual decommissioning. The ISPE AI maturity model and FDA 7-step framework both emphasize continuous monitoring, validation, risk assessment, and transparency, with the ISPE model focusing on autonomy and control levels, which align with the FDA’s steps for monitoring, validation, and ongoing evaluation.
To facilitate this, sponsors can implement robust AI policies that accommodate the dynamic nature of AI systems. This includes establishing clear protocols for reporting any changes that impact model performance and ensuring that AI systems remain part of the manufacturer’s pharmaceutical quality system. These actions help ensure that AI models meet regulatory expectations and continue to operate effectively and safely throughout their life cycle.
Ensure Continuous AI Model Performance Integrity Throughout Drug And Biologics Development Life Cycle
Traditional regulatory models assess safety and functionality at fixed points in a product’s life cycle, requiring incremental updates based on data. However, these models are less suited to managing the dynamic and iterative nature of AI/ML technologies, which can evolve autonomously through self-learning mechanisms. While AI systems enhance real-time optimization, they also introduce challenges related to diminished human oversight, unforeseen risks from data adaptation without reevaluation, and ethical concerns regarding biased or poorly labeled data. For high-risk applications, such as patient stratification in life-threatening conditions, additional guidance on real-time monitoring and fallback mechanisms is essential to ensure safety and prevent unintended consequences. Ongoing validation and monitoring are critical to detect performance degradation early, ensuring AI models remain reliable and adaptable for regulatory decision-making. Maintaining model performance integrity across the entire product life cycle is crucial to maximizing AI’s potential in drug development, from discovery through post-market surveillance.
Improve Predictions Over Time In Dynamic AI-Driven Drug Development
Statistical predictive models offer an adaptive and flexible approach for continuous oversight in drug development. These models excel in handling incomplete or noisy data, enabling AI systems to improve predictions over time. Both the EMA and FDA emphasize the integration of advanced statistical tools and the inclusion of RWD and RWE in AI models, particularly for small patient populations or rare diseases where traditional clinical trial designs may fall short.1,2,6-8 By combining statistical models with RWE, the FDA and sponsors can gain deeper insights into drug performance across diverse populations, accelerating approvals and optimizing regulatory processes.
Drug Discovery
AI has the potential to accelerate drug discovery by integrating large-scale pharmacological and genetic data, identifying promising drug compounds, and even repurposing existing drugs. AI can improve quality by design approaches, particularly by enhancing the interpretation of big experimental data from sources like real-time process control and quality assurance.9 Early identification of effective compounds can significantly shorten development timelines and reduce the costs associated with traditional trial designs.
AI and machine learning (AI/ML) typically have lower regulatory oversight in drug discovery unless their performance directly impacts regulatory decisions. However, when AI/ML-driven results contribute to regulatory evidence, it’s essential for sponsors to thoroughly assess all models and datasets for potential risks. This evaluation is vital for developing risk-mitigation plans, particularly concerning data quality, quantity, and ethical concerns during data collection and model training.
Non-Clinical Testing And Toxicity Prediction
AI plays a key role in predicting toxicity during the non-clinical phase by utilizing toxicological big data. Models such as RASAR (Risk Assessment of Synthetic Alternatives for Replacement), powered by ML, allow for more accurate toxicity predictions and animal testing reductions.10 By integrating AI into toxicity prediction, new regulatory standards may emerge, especially for the use of non-animal testing methods. This is critical in developing safer drugs faster.
Bayesian models also offer critical insights during drug development’s early phases, particularly in pharmacokinetic (PK) and pharmacodynamic (PD) modeling. These models can be used to optimize dosing schedules, identify biomarkers, and assess drug safety and efficacy more efficiently, especially when clinical trials face challenges due to small sample sizes or complex disease states.6-8
Translational And Clinical Research: Optimizing Trials
In the clinical phase, AI models can refine patient selection, optimize clinical trial designs, and predict outcomes by incorporating RWD and RWE.11,4 The integration of synthetic data and digital twins can enhance trial design, and AI systems can assist in validating models by ensuring synthetic data reflects real-world conditions. This enhances clinical trial efficiency and speeds up the approval process.
For example, Bayesian modeling enhances post-market risk assessments, ensuring patient safety while reducing unnecessary drug restrictions. They are particularly effective in addressing data quality issues and enhancing model generalizability. In rare pediatric diseases like Duchenne Muscular Dystrophy (DMD), Bayesian simulations combine study data to predict outcomes, optimize trial designs, and maximize limited data.12, 6-8 This ensures that AI systems remain effective and that new risks are mitigated. Continuous monitoring of AI models is critical in ensuring they remain adaptable to new data over time, especially in evolving environments, particularly in rare diseases, urgent clinical contexts, and when traditional clinical trials are difficult to implement.
Production Line QC And QA
With the rise of continuous manufacturing, AI is essential in ensuring compliance with regulatory standards. AI-driven quality control (QC) and quality assurance (QA) models are helping pharmaceutical companies streamline manufacturing.13,14 Traditional QA struggles with incomplete, biased, or inaccurate data, affecting model reliability. Manual testing cannot scale to handle large datasets and complex AI requirements, and human biases or fatigue can compromise efforts. AI-driven QA tools, however, autonomously create test cases from natural language inputs, reducing manual effort and enhancing consistency across rapid development cycles. Models for process design and optimization are now used to monitor production quality in real time.
Pharmacovigilance And Post-Market Surveillance
In the post-market phase, AI is critical for monitoring drug safety, detecting adverse drug reactions (ADRs), and ensuring long-term safety.7-8, 11 Through the integration of RWD, including HER, AI is improving pharmacovigilance by identifying safety signals earlier and more effectively. ML algorithms can process large datasets and detect potential issues before they escalate.
Global Alignment And Ethical Considerations
As AI continues to shape drug development, the FDA’s regulatory framework must align with international efforts, such as those by the EMA and CDE in China. Global collaboration will help standardize AI usage in drug development and regulatory decision-making. The FDA should work with global agencies to harmonize data use from international trials and ensure consistent ethical standards for AI. Furthermore, AI models must be transparent, and data used in training should be diverse and free from bias to maintain trust and regulatory compliance.15
AI’s potential in clinical trials and post-market surveillance is vast, particularly when integrated with RWE. Global regulatory efforts are recognizing the value of RWD and AI in clinical trials. The FDA, EMA, and other agencies are working toward harmonizing AI frameworks to enable better data usage across global trials.15 Collaboration between global regulatory agencies is key to streamlining multinational trials, reducing duplication, and ensuring that AI models remain robust and generalizable. Creating consistent frameworks across markets is essential for harmonizing international regulations and facilitating broader adoption by multinational sponsors.
Conclusion
Continuous oversight and model integrity are essential to unlock AI’s full potential in drug development. By combining the FDA’s 7-step framework with dynamic life cycle management, AI systems can be consistently validated and recalibrated throughout their life cycle. Ongoing monitoring, transparency, and accountability will ensure that AI systems remain compliant, adaptable, and reliable, safeguarding patient safety. As AI continues to evolve, effective regulatory collaboration and continuous validation will enhance efficiency and benefit public health, ensuring AI’s responsible use in drug development.
References:
- FDA, U. Considerations for the Use of Artificial Intelligence to Support Regulatory Decision-Making for Drug and Biological Products. Guidance for Industry and Other Interested Parties DRAFT GUIDANCE 2025.
- NIST, AI RISK MANAGEMENT FRAMEWORK, 2024. Available from: https://www.nist.gov/itl/ai-risk-management-framework.
- EMA. White Paper on Artificial Intelligence: a European approach to excellence and trust. 2020. Available from: https://commission.europa.eu/document/d2ec4039-c5be-423a-81ef b9e44e79825b_en.
- FDA, U. Real-World Data: Assessing Electronic Health Records and Medical Claims Data to Support Regulatory Decision-Making for Drug and Biological Products: Guidance for Industry. 2024.
- ISPE ISPE Quality Management Maturity Program: Advancing Pharmaceutical Quality, 200. Available from: https://ispe.org/pharmaceutical-engineering/ispeak/ispe-quality-management-maturity-program-advancing-pharmaceutical.
- FDA, U. Guidance for the Use of Bayesian Statistics in Medical Device Clinical Trials. 2010. Available from: https://www.fda.gov/regulatory-information/search-fda-guidance-documents/guidance-use-bayesian-statistics-medical-device-clinical-trials.
- Kidwell, K.M., et al., Application of Bayesian methods to accelerate rare disease drug development: scopes and hurdles. Orphanet Journal of Rare Diseases, 2022. 17(1): p. 186.
- Ruberg, S.J., et al., Application of Bayesian approaches in drug development: starting a virtuous cycle. Nature Reviews. Drug Discovery, 2023. 22: p. 235 - 250.
- Manzano T, Fernàndez C, Ruiz T, Richard H. Artificial Intelligence Algorithm Qualification: A Quality by Design Approach to Apply Artificial Intelligence in Pharma. PDA J Pharm Sci Technol. 2021 Jan-Feb;75(1):100-118. doi: 10.5731/pdajpst.2019.011338. Epub 2020 Aug 14. PMID: 32817323.
- Luechtefeld T, Marsh D, Rowlands C, Hartung T. Machine Learning of Toxicological Big Data Enables Read-Across Structure Activity Relationships (RASAR) Outperforming Animal Test Reproducibility. Toxicol Sci. 2018 Sep 1;165(1):198-212. doi: 10.1093/toxsci/kfy152. PMID: 30007363; PMCID: PMC6135638.
- FDA, U. AI and ML in the development of drug and biological products, 2023.
- Wang, S., K.M. Kidwell, and S. Roychoudhury, Dynamic enrichment of Bayesian small-sample, sequential, multiple assignment randomized trial design using natural history data: a case study from Duchenne muscular dystrophy. Biometrics, 2023. 79(4): p. 3612-3623.
- Ogrizović, M., D. Drašković, and D. Bojić, Quality assurance strategies for machine learning applications in big data analytics: an overview. Journal of Big Data, 2024. 11(1): p. 156.
- Mahmood, U., et al., Artificial intelligence in medicine: mitigating risks and maximizing benefits via quality assurance, quality control, and acceptance testing. BJR|Artificial Intelligence, 2024. 1(1).
- Schmallenbach, L., T.W. Bärnighausen, and M.J. Lerchenmueller, The global geography of artificial intelligence in life science research. Nature Communications, 2024. 15(1): p. 7527.
About The Author:
Fahimeh Mirakhori, M.Sc., Ph.D. is a consultant who addresses scientific, technical, and regulatory challenges in cell and gene therapy, genome editing, regenerative medicine, and biologics product development. Her areas of expertise include autologous and allogeneic engineered cell therapeutics (CAR-T, CAR-NK, iPSCs), viral vectors (AAV, LVV), regulatory CMC, as well as process and analytical development. She earned her Ph.D. from the University of Tehran and completed her postdoctoral fellowship at Johns Hopkins University School of Medicine. She has held diverse roles in the industry, acquiring broad experience across various biotechnology modalities. She is also an adjunct professor at the University of Maryland.