AI Model Cards Make Function And Risk Easier To Understand
By Andy O’Connor, ERA Sciences
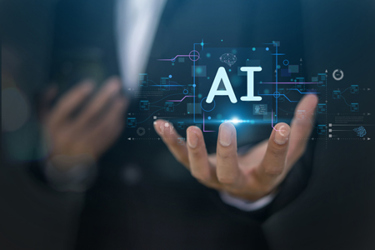
Artificial intelligence is shaking up the pharmaceutical industry, delivering efficiencies in manufacturing, quality control, and real-time monitoring. But with great potential comes great responsibility — especially in regulated environments. For us IT and quality directors, ensuring patient safety, product quality, and data integrity is not negotiable. The challenge? AI is moving faster than compliance standards, leaving many unsure of what they need to do.
AI models don’t follow predefined logic like traditional software. They’re non-deterministic, operating on probabilities, which presents a regulatory puzzle. Traditional validation frameworks like GAMP 5 and requirements such as 21 CFR Part 11 and Annex 11 can still apply. Impact assessments, business requirements, and risk evaluations are all still relevant; however, traditional positive, negative, and boundary testing often used as forms of risk mitigation may no longer be appropriate.
AI throws in new concepts to consider, like bias assessments, statistical error rates, and monitoring for false positives and negatives. When performing quality assurance on an AI model, it raises new questions for us:
- How well does the model predict quality, and is it actually better than a non-ML approach?
- What is the statistical error rate or what statistical documentation can you provide from evaluation?
- What are the model limitations?
- What data has it been trained/tested/validated on?
- Is the data of high quality?
- Who labeled the data?
- Has the data been checked for biases, including societal inequities (think clinical trial representation)?
- How are outliers handled?
- What are the consequences of false positives/false negatives?
- Does the model use static or dynamic training?
- How do you feed deviations back into the model?
- What is the business?
Datasheets and labels are already a standard way to document warnings and limitations in pharma. Model cards apply the same logic to AI, providing a streamlined way to answer critical compliance questions. Here’s a model card specifically designed to support fast QA and compliance feedback:
Model cards give a quick yet comprehensive view of a model’s intended use, limitations, and risks. They ensure regulatory teams have the details they need to evaluate AI’s impact. More than just documentation, model cards establish transparency in decision-making and act as a single source of truth throughout the model’s life cycle. They empower IT and quality directors to make informed risk assessments without getting lost in technical jargon.
First and foremost, the model card bridges the chasm between technical engineering and quality assurance for an effective determination of quality and decision-making. Where AI outputs have a direct and critical contribution to a regulatory submission, including AI model cards is an effective way to anticipate and address any regulatory concerns and reduce any delays. Simplifying the purpose, function, and risk of a model into a structured format helps satisfy what regulators are looking for: clarity, explainability, and traceability.
By bridging the gap between AI development teams and quality assurance personnel, model cards turn machine learning complexity into actionable insights. They enable risk-based decision-making and instill best practices in AI governance, ensuring models stay auditable, explainable, and GxP-compliant.
Model cards make compliance easier, offering a structured yet flexible way to evaluate an AI model’s reliability, safety, and compliance at a glance. To simplify AI compliance, ERA Sciences has developed a ready-to-use model card template aligned with industry best practices.
Click here to download a template kit.
A version of this article first appeared on ERA Science’s blog. It is republished here with permission.
About The Author:
Andy O’Connor is a director at ERA Sciences with over 16 years of experience in risk management and technology within the life sciences industry. He focuses on quality and IT governance for companies transitioning from clinical to commercial manufacturing. He holds an honors degree in science from University College Dublin. As a software developer, Andy has contributed to numerous enterprise application projects and hosts client training events and workshops on risk and data integrity.