AI Beats Back Bad Batches

By Louis Garguilo, Chief Editor, Outsourced Pharma
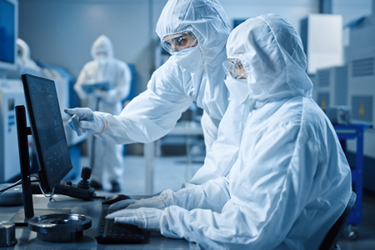
I induce the estimable Professor Tinglon Dai of Johns Hopkins University, an acclaimed AI specialist in the field of supply chain, to laugh aloud a few moments into our conversation.
“So,” I say, “I’m talking to a renown AI supply-chain expert, and the first solution for improving drug development and manufacturing outsourcing he suggests is RFID tags?”
The professor may be all about the advancing of artificial intelligence applications in the biopharma industry, but he’s devoted to bringing forth practical business solutions. He’s fully cognizant of the costs of any suggested AI solution, and prefers to focus on those currently implementable.
“I look from a supply-chain perspective,” Dai says, “discovery, development, the manufacture of products, until we actually put them into clinical use.”
His highest mission for AI implementation is also our highest – improved drug/employee/patient safety, the following of cGMP protocols and regulations, and the prevention of errors.
And that involves, he says, “keeping track of multiple stakeholders.”
AI AND RFID
Dai holds numerous positions simultaneously at Johns Hopkins. He is regularly interviewed and quoted in various news media, from the Financial Times to Fortune and PBS.
“AI-machine learning,” he often says together in rapid succession, as if one word, can be useful in the overall creation of drugs and therapies “for the visibility it can provide into what's really going on at the plant-floor level, and for the coordination of activities” within and throughout the supply chain.
As example, he points to the problems that occurred at a CDMO attempting to produce two vaccines during the early days of COVID.
Multiple and valuable batches had to be discarded primarily because of fears of cross contamination from one production line to the second. “It resulted in a significant waste of taxpayers money,” Dai says, not to mention other negative impacts on the entire vaccine-creating effort.
“So what happened in that case?” he asks rhetorically. “We now know at issue was a production worker servicing both production lines – one for J&J and one for AstraZeneca – was the contaminator.”
“When you physically move from one such GMP product line to another, generally speaking, you need to shower or follow other hygiene protocols. Those protocols were not adhered to. This is something that could have been easily detected through a very simple algorithm set for the amount of time activities normally require to be completed."
Employee tracking can be accomplished through inexpensive means, such as radio frequency identification (RFID) tags – on the market and in use for decades – embedded in employee badges, and that send data to an AI program.
“When an AI program, for example, looks at time stamps of a person moving from one line to another, if that duration is too short, it’s something really basic that can be flagged immediately," says Dai. “At a fundamental level, this is simply giving an organization better visibility, early warnings, and continuous monitoring and analysis as to what's going on with each individual operator.”
RFIDs, Dai points out, are already used in hospitals, for example to study patient flow.
Yes, it’s a" low-tech solution," but coupled with AI it's a continuous-monitoring data stream sent to a server with capabilities to identify the correct sequence of activities, the time to accomplish them, and the ability to detect anomalies that trigger warnings to managers and supervisors. And no human resource is necessary until that manager or supervisor is alerted.
Where’s The Data?
Since we are talking about CDMOs, I ask Dai for details on the server collecting data on client projects.
Should the server/data be locally situated? Is the “cloud” or third-party storage safe? Do clients or regulators get access?
Of course this question is not specific to RFID-tag generated data. Streaming cameras are already ubiquitous in areas of our industry as tools for machine, material, packaging, storage, and employee monitoring. So my question is where does the AI analysis of that video or those images take place?
Dai believes to start, the CDMOs should host these AI-enhanced servers on-site. Also, in most all cases, he believes, the data does not have to be transferred externally.
“These manufacturers work with multiple clients, so yes, they need to determine how best to safeguard their clients’ IP and scientific information,” he says, while internally utilizing learnings. That means safeguards need to be established by the CDMO.
“However,” he quickly adds, “if we are talking in terms of this solution as a manufacturing practice to reduce the chance of contamination – contamination quality issues contribute to about 60% of all drug shortages – it is well worth the effort.”
“An RFD solution or camera’s throughout the facility feeding images into AI programs should be something you can get all your various stakeholders on board with.
“It’s an opportunity to collectively, throughout the industry, save billions of dollars of waste because of contamination, operator error, and ultimately enhance worker and patient safety. It would definitely involve some in-house implementation, human and financial resources, but this is a reasonable and comparatively inexpensive implementation.” (see part one)
Furthermore, as an industry, we should come to some terms for when AI-machine learning should in fact be shared. Dai believes by doing this, we will actually build further trust among CDMOs, sponsors, and regulators. Everyone gets more visibility into and assurance of what is transpiring within the walls of labs and plants.
Global Reach
The above example of contamination happened at a U.S.-based facility. Dai next refers to a more recent episode at a manufacturer in India, one that directly contributed to a drug shortage here in the U.S.
“Basically,” he says, “we have no idea what happened in this particular Indian factory. All we have is a report from the FDA, but that report doesn’t provide us much insight, the full extent of the problem, or the general quality of the manufacturing practices."
In fact, Dai reminds me, “We would not have known anything if the FDA had not performed an unscheduled audit of the facility in India in the first place.”
Which begs the question:
Should regulatory bodies, perhaps led by the FDA, begin to look into requiring that manufacturers utilize RFID/AI-like solutions to monitor and analyze workflow and workplace variables, and alert managers when anomalies occur?
If so, those decisions on where/how the data is stored, and who gets to look at it should problems occur, will need to be addressed. The industry ecosystem will need to come together, and ultimately it will be for the benefit of safer medicines and patients.
There certainly will be more “high-tech solutions” for future consideration, such as those we associate with the internet of things (IoT), and what we refer to as Biopharm 4.0.
But globally implementable AI-machine learning solutions are available right now. What are we waiting for?
----
Part one in this series is here: AI, The Ghost In The Outsourcing Machine