Not Enough Data To Utilize AI And Machine Learning In Drug Development

By Louis Garguilo, Chief Editor, Outsourced Pharma
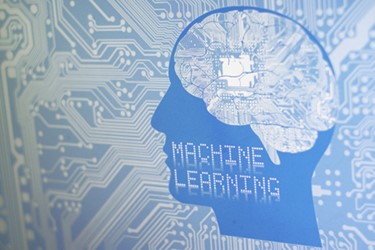
We’ve heard the calls for help: “We’re drowning in oceans of data.”
But I’ve learned that those further “downstream” may end up high and dry. Particularly when it comes to having sufficient data to allow drug developers and manufacturers to fully actuate artificial intelligence (AI) and machine learning (ML), in the pursuit of improving production processes and productivity.
Discovery Data vs. Development Data
Meet Stephen Harrison, CSO & SVP, Engine Biosciences. He’s got a Ph.D. from the University of Cambridge, a post-doc from Berkeley, years of experience at various biopharma organizations … and plenty of data for the drug discovery activities he and his company undertake utilizing advanced – and some proprietary – AI/ML tools.
But when he considers biopharma companies and their CDMOs attempting to advance AI/ML to development and manufacturing, he sees a dearth of data as a limiting factor hard to overcome.
“That is the crux of the discussion today,” he said as a panelist at a recent Outsourced Pharma San Francisco conference. “Will we be able to collect enough data? Is it, for example, possible that any one CMO – or even any one pharma company – will obtain sufficient data to really take advantage of these artificial intelligence tools?”
The answer right now appears to be no. Harrison contrasts this with what’s available on the discovery side. Engine, based in Singapore, starts by mining the vast, rather readily available amounts of human-clinical data being generated today, particularly those data sets incorporating vast amounts of genomic information, as well as data on the transcriptional state of cells, and much more.
Within that explanation, he explains the need for (human) formation of hypothesis to spur the tools of analysis and learning. And he describes “another caveat that will surely spill over to the development side: the validation of any findings.”
“Companies believe they can use artificial intelligence and machine learning tools to look through this vast amount of data and be able to identify critical disease pathways worth intervening in. But any in silico approach is mostly going to be correlative. You will be looking for statistical associations …and you need to have real world validation,” Harrison explains.
“To really use true machine learning, it’s vital to have lots of data that helps us understand the outcome of our predictions, and to be able to feed that back to the starting point. That is in our experience in the early stage. The limitation we will face downstream is having enough data to do any of that.”
Can We Get More Data?
The data that does exist in later-development and API/product manufacturing – as it always has – resides locked within individual pharma companies. Are they ever going to release or pool that data with other pharma and CDMOs? While that seems unlikely, perhaps circumstances will change when they learn their ability to unlock the potential of AI/ML in those sectors of the supply chain will be severely limited if they don’t.
Of course CDMOs and other later-stage service companies generate a lot of data as well, but most of that, too, is customer-protected IP. And then there’s that little problem of having a business model based on an inherent and strict code of secrecy. That model rarely if ever allows for the combining of enough data from enough customers, with enough drug development projects and products, to reach the amounts of data for the AI/LM iterations needed to take advantage of these tools.
Rick Panicucci, SVP of CMC of biotechs QED Therapeutics and Origin Biosciences, and who has also worked on the CDMO side, joined Harrison on the Outsourced Pharma Panel. “I do see that some biopharma companies are starting to at least discuss the topic,” he said. “I know of some companies specifically that have made it part of their corporate objectives to seriously look into these technologies and data share. Still, it takes a lot of data from a lot of activities you perform, and the question remains: ‘Do I want my data to go into a database that then helps my competitor develop a better process?’ So there are factors we will have to work through, but it’s clear this is a direction we are at least thinking about.”
A third panelist, Bikash Chatterjee, President & CSO, Pharmatech Associates, used Shire as an example of tangible progress. “Shire, a one-hundred percent outsourcing model, is fundamentally using a shared database across all their CDMOs, for the intent of improving performance across each. It’s an extraordinary situation to imagine one CDMO helping another get better at what they do, but that is the end game if you are going to use an outsourcing model where all of your contract service providers are collaborating at some level to drive increased performance for your products as you move forward.”
Chatterjee continued: “Regarding whether AI or machine learning can leverage that, I’d say rather we are more likely at the data generation and acquisition stage in terms of that particular evolution. But we are moving in the right direction. There is more data out there if we can only bring it together.”
A Consortium In Our Future?
One suggestion for bringing more data together is the creation of neutral consortia.
These organizations would be established specifically to house data from the industry, and via some form of deconvolution and IP protection, allow biopharma and their contract developers and manufacturers to run AI/ML tools with the pooled data sets. This model was proposed in the EU as part of a similar problem to address the EU’s clinical data transparency directive in 2013.
Mark Butchko, Senior Director, Quality Assurance – Development, Eli Lilly and Company, said this from the session audience:
“I did not think we would one day see Big Pharma competitors running combination studies or collaboratively like we do today. I did not think we would be talking about consortiums like TransCelerate, where one pharma company can collaborate as part of the consortium, and maybe even go through a common outsourcing partner to provide access to comparator drugs from one pharma company to another. So, I would not necessarily say Big Pharma has all this data, but they are unwilling to share it, because I think we’ve changed the winds of partnership and collaboration within the industry in the last five or ten years. Probably immuno-oncology is much to thank for that.”
Good to hear … but we’ll give the last word back to Harrison:
“Unfortunately, I would even question that with Big Pharma and CMO data freed up, and consortium created, there would still be enough data to really take advantage of AI/ML.”
It seems for some scientists, there’s just never enough data.
---------
This editorial was based on the Outsourced Pharma San Francisco 2018 session, “How To Think About Data, AI … And Human Knowledge,” moderated by Ravi Kiron, Head, BioPharma External Innovation, EMD Serono. Panelists were:
- Bikash Chatterjee, President & CSO | Pharmatech Associates
- Kumar Gadamasetti, CEO | Certum Pharma/Biotech
- Stephen Harrison, CSO & SVP | Engine Biosciences
- Rick Panicucci, SVP of CMC | QED Therapeutics