How Modeling Predicts Bottlenecks In Takeda's Multimodal Facilities
A conversation with Minhazuddin Mohammed, Takeda
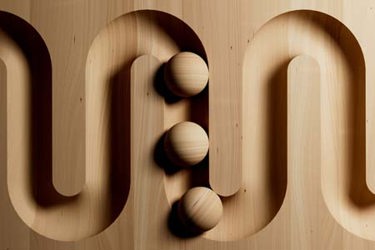
Resource coordination presents an obvious use case for model simulation, and few applications illustrate the breadth of its potential more than multimodal manufacturing.
But getting to a functional simulation requires many manual inputs, including smoothing out inconsistent data feeds, close collaboration with vendors, and even oral history from people who have been doing the work the longest.
A team at Takeda’s Massachusetts Biologic Operations site explored what it takes when they built a virtual model to predict conflicts and bottlenecks. Minhazuddin Mohammed, senior manager of process engineering at the site, was part of the effort, and he’s slated to speak about their work during the International Society for Pharmaceutical Engineering’s 2025 ISPE Biotechnology Conference.
He offered to give us a preview and answer questions about what they learned.
What modeling framework or engine are you using to simulate your site? And how customized is it for your specific needs?
We use SchedulePro as the core simulation app to model our plant that manufactures multiple products of different modalities via interconnected and disconnected suites. The software provided a solid foundation, but several critical customizations were necessary to truly reflect the operational realities of our site.
For example, standard functionalities could not initially capture nuances like suite sharing constraints between different products while allowing different batches of the same product, selection of a single transfer panel from a shared pool, or triggering column packing activities based on resin cycle counts. These limitations posed risks to accurately forecasting throughput and resource utilization in our specific environment.
To address this, we partnered directly with the vendor to enhance the software. New features were developed to better model the unique constraints and tribal practices of our site. This development approach allowed us to progressively increase model fidelity and enable simulation outputs that better reflect the complexities of our plant.
Can we talk about data hygiene? So often, unstructured or incompatible data feeds create major bottlenecks. Is this a problem for a methodology that relies on data from multiple products and teams?
Data hygiene was absolutely a major challenge for us and one of the most time-consuming parts of building the model. Much of the critical information lived across disconnected systems, and reconstructing a complete, reliable operational picture required extensive research across multiple sources. What made this process smoother was the fact that the core team, including myself and other SMEs, have deep historical knowledge of the site. We understood not just where the data lived but also the undocumented nuances behind the numbers — the tribal knowledge that allowed us to assess whether the data made sense or needed deeper investigation.
To improve consistency, we made it a point to centralize all input data we used — for example, archiving trend charts for durations, flow rates, and cycle times in a shared repository that allowed us to back-check assumptions as the model evolved.
Even with these strategies, data gathering remained a significant effort. Ultimately, it was a combination of leadership support and personal commitment that allowed us to push through the complexity and ensure the model was grounded in credible operational reality.
How do you predict shared resource utilization and the inherent variability of human-run processes? Are you modeling deterministic or stochastic demand?
Our initial focus was on deterministic simulation — creating best-estimate timelines based on planned production sequences and the long-range production forecasts provided by our global supply chain teams. Starting with a deterministic approach allowed us to quickly establish a baseline understanding of our site's capacity, identify short-term improvement opportunities, and prioritize tactical actions without the additional complexity of uncertainty modeling.
To account for the variability within this deterministic framework, we have set an internal 85% utilization acceptance threshold for our primary bottleneck unit operations. This gave us a practical buffer to accommodate some variability without grossly overestimating or underestimating our capacity and future capabilities. The deterministic model has also been helping us project when future bottlenecks are likely to emerge along our long-range plan horizon and align capital investment strategies to address them in a phased, informed manner.
Important to note here is that because of the model’s current scale and complexity, individual simulation runs can take two to three days to complete. Running full Monte Carlo simulations would exacerbate these runtimes significantly. As a result, we are actively working to simplify and consolidate aspects of the model where appropriate, creating a leaner structure that will allow us to explore variability more efficiently without sacrificing operational fidelity. That said, we fully recognize that manufacturing variability — including equipment failures, batch contaminations, and biological process variability (such as cell expansion timing based on viable cell density triggers) — plays a significant role in optimized planning. We intend to layer variability analyses of these factors onto the model in future phases to better quantify our capacity and planning risks.
How does the model inform real-time decision-making — like in response to equipment failure?
Today, our capacity model is primarily used for strategic and tactical planning rather than direct real-time schedule adjustments. While we have not yet incorporated full variability analyses, the operational breathing room we established — including limiting bottleneck utilizations to approximately 85% — provides flexibility to absorb common disruptions, such as equipment failures. When disruptions do occur, we use the model after the fact: supply chain and operations teams can reach out to quickly simulate replan scenarios, enabling us to realign subsequent campaigns and minimize cascading delays.
Looking ahead, our vision is to evolve toward a true living capacity model — one that can rapidly simulate alternative paths in response to events like unexpected downtime or supply chain disruptions. We intend to integrate the existing model into a production server environment or feed its logic into a real-time finite scheduling application, allowing operations teams to test and select feasible recovery options before finalizing rescheduling decisions. While the choice of real-time scheduling application is still under evaluation, our capacity model is intentionally built to support this transition without requiring major redevelopment.
What happens when the product mix changes? How well does the model receive new data inputs?
Product mix changes can happen in two ways: either through shifts in campaign cadence among existing products or through new product introduction (NPI) that alter the manufacturing portfolio.
If a disruption occurs in the planned cadence — for example, if batch volumes shift or scheduling priorities change — supply chain or planning teams can reach out to us, and we can rapidly simulate different replan scenarios to assess impacts on the site's volume requirements. It’s important to note that supply chain maintains a separate scheduling tool for daily manufacturing planning, whereas our capacity model is designed specifically to analyze capacity bottlenecks from a strategic and tactical perspective. As mentioned previously, we are not yet configured to automatically receive real-time process or supply changes, so model results are not updated in real time; instead, we work offline to manually explore replan scenarios as needed.
When a new product is introduced to the facility, the existing recipes and preconfigured facility data in the model allow for relatively quick onboarding of the new process. This typically involves:
- adding new recipes and materials using existing templates and updating unit procedures as needed, and
- if a new constrain is identified and the software cannot model it, we identify a temporary workaround while working in parallel with the app vendor to get the software updated.
What lessons would you share with other facilities trying to move from static models to continuous operational modeling?
The biggest lesson we learned is that continuous operational modeling is a long-term commitment, not just a technical project. Early awareness of the time investment and computational feasibility constraints is critical — it helps set realistic expectations for building and maintaining a living model. Equally important is securing leadership sponsorship at the outset. The organization must see the strategic value of a living capacity model, not just as a planning tool but as a core operational asset that enables better decisions over time.
Once the model is validated, it’s essential to integrate it into formal governance processes — such as change control. Any process, facility, or material change that impacts model inputs must trigger an assessment of whether the model needs to be updated. Embedding model assessment directly into routine change control workflows ensures the model stays aligned with operational reality.
Sustaining a living model also requires establishing a trained, accountable team. Model updates should be performed by qualified personnel following preapproved instructions under a governing procedure, ensuring that assumptions, constraints, and modeling methodologies are consistently documented and traceable.
Finally, to fully realize its potential, the model must be marketed internally — promoted as a trusted tool for answering what-if questions and scenario analyses. By embedding the model into the site's culture and daily decision-making, it becomes approachable, familiar, and ultimately indispensable to sustaining operational excellence.
About The Expert:
Minhazuddin Mohammed is the senior manager of process engineering at Takeda’s Massachusetts Biologic Operations site and an active member of ISPE. He leads a team of bioprocess engineers responsible for designing, operating, and continuously improving biomanufacturing equipment. Before Takeda, he held roles at DuPont, Merck, and ValSource, including R&D process engineer, investigator, validation engineer, and quality assurance specialist. He earned bachelor’s and master’s degrees in chemical engineering from Drexel University. He specializes in capacity modeling, equipment design, and techno-economic feasibility to tackle challenges in multi-product biomanufacturing.