5 Characteristics Of The Automated Microbiology Lab Of The Future
By Tim Sandle, Ph.D.
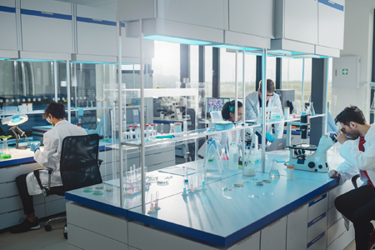
The modern microbiology laboratory is starting to look very different from the laboratory of 10 years ago, and in the next few years, the microbiology laboratory is set to appear different from the laboratory of today. The work will still involve assessing microbial numbers, determining susceptibility, and identifying organisms, and there will continue to be laboratory analysts (albeit fewer in number), yet technology will have advanced. Central to this advancement are automation and artificial intelligence.
At one level, attempting to predict the future course of technology is challenging since developments often take unexpected turns; equally, there are a number of innovations being implemented now or are in progress that can signal the path that many microbiology laboratories will follow. This article considers a selection of these.
Implementation Of Automated Processes In The Lab Workflow
Automating the laboratory brings many advantages: a reduction in errors and greater data insights. The laboratory of the future will be based on cloud-based laboratory management and automation platforms. The extent of automation is unclear given the plethora of complex multicomponent systems. Yet based on what is currently available, there are considerable advantages of automation.
At the simplest level, laboratory automation exists to remove the more repetitive tasks, such as performing dilutions or plate spotting. At a more advanced level, entire workflows can be automated, as with the case of robotics designed for bacterial endotoxin testing. In between are automated workflows to manage complex tests, balance multiple workflows, and reduce the most time-consuming steps, such as sample preparation.
Three current examples in the microbiology laboratory are:
- Reproducibility: Reproducibility is a common concern in microbiology, especially for techniques reliant upon the variances of laboratory analysts. Examples of automation technologies that can drive improvements include robotics for streaking samples onto the agar plates and inoculating liquid culture media. These forms of laboratory automation can enable a greater rate of experimental data capture, increased volume of results, and the use of a wider range of controls.
- Traceability: Sample traceability is important for business efficiency, and it is a core part of cGMP. The primary way traceability is automated is with the bar-coding of samples. Automated solutions connect bar code scanners, printers, plate readers, incubators, test devices, and information management solutions.
- Data accuracy: Data accuracy is essential, not only to meet cGMP requirements but also to ensure appropriate insights can be drawn. A new array of automated processes are incorporating machine learning algorithms that strengthen the insights from data evaluation. These technologies become more sophisticated by learning from large pools of real samples.
In terms of automation advances and what the microbiology laboratory of the future is set to exploit, let’s look at five key opportunity areas.
1. Biosensors
In the areas of drug development and disease diagnosis, lab-on-a-chip technology holds the potential to move laboratory analyses away from traditional diagnostic platforms to more powerful technology. Biosensor devices consist of small chip-based miniaturized platforms that can be used for the immediate detection of different organisms and to assess how they interact with different compounds, by using analytes of low volumes. These devices are based on microfluidic technologies. Microfluidic devices work by assessing the physical and chemical properties of liquids at a microscale, enabling dozens of tests to be conducted simultaneously.1
A related development has seen the creation of a microfluidic fluorescence quantitative PCR system with a pneumatic valve and a tree structure that was developed by using 3D printing technology. This is necessary because the objective is to create well-defined microenvironments that mimic the natural habitats of bacteria.2
2. Assessing Microbial Colonies Early
The majority of methods within the microbiology laboratory are growth-based, reliant upon agar or broth culture media. It is often the case that obtaining a result as fast as possible aids in faster reporting and release of product. Several technologies are focused on providing a faster reading of agar plates. Examples include the use of light forward-scattering and learning algorithms3 and digital imaging of cellular autofluorescence to detect and enumerate growing microcolonies many generations before they become visible to the eye.4
Where multiple incubation steps are required, some systems have the ability to automatically transfer culture media to specialized incubators operating at different temperature ranges through robotic systems.
An alternative option is the array of automated colony counting technologies available.5 Some of the technologies have struggled to accurately count mixed cultures, although improvements have been made with innovations like hyperspectral imaging technology.6
3. Microbial Identification
MALDI-TOF has become the identification method of choice in many laboratories, and it has drastically cut down preparation time and time-to-result.7 In terms of future developments with identification technology, there is considerable interest in surface-enhanced Raman spectroscopy (SERS) analysis. This is used in conjunction with a deep learning model, based on optimizing the route to classify the signals of two common bacteria and their resident media without the need for any separation procedures. This form of Raman spectroscopy functions by sending light through a sample to observe how it scatters.8 Current developments indicate it is possible to classify various bacteria in different media with accuracies of up to 98%. The algorithm is based on the functionality of a dual-branch wide-kernel network and it is trained to reveal and assess structural information about a given sample (the spectral fingerprint).
There is an associated benefit in relation to drug development where convolutional neural networks (CNN) can be trained to classify noisy bacterial spectra by isolate, empiric treatment, and drug resistance. Hence, such technology can assist with developing susceptibility profiles.9
4. Transfer Of Data To LIMS Or Equivalent Systems​
To reduce data errors and to speed up the analysis process, the smart microbiology laboratory will seek to connect as many instruments to laboratory information management systems (LIMS) as possible.10 As microbiology equipment progresses, the required interfaces between the equipment and LIMS is made possible. One example is the connection of microbial identification systems like MALDI-TOF MS into these workflows.
To accommodate advances in laboratory analytical equipment and to enable fuller insights to be gained from data, LIMS technology needs to advance. LIMS is often hampered by limited database flexibility owing to instrumentation, insufficient traceability, inadequate data protection, and low management efficiency. These obstacles are being overcome by projects seeking to construct smart central management systems modeled on the human brain based on the LIMS; that is combined with current advanced technologies, such as information modeling and artificial intelligence.11
Many of the elements of laboratory automation can support this next-generation LIMS, including high-definition cameras, sensors, automatic control units, and robotic arms.
5. The Use Of Artificial Intelligence To Analyze Data
Artificial intelligence (AI) has the potential to analyze Big Data and find patterns and insights that could enhance understanding microbial growth, improve microbial identification, enable using microorganisms to produce new compounds in biotechnological applications, and assist with antimicrobial drug development. In each of these cases, processing data becomes much faster and more meaningful patterns can be captured. Giving any individual many different data points without synthesizing and analyzing the information makes it difficult to comprehend the information. AI can conduct the analysis and provide real insights, direction, and even advice. It is likely that developments within the pathology sector will provide the basis for pharma. The FDA has approved a digital pathology system, an artificial intelligence platform called OsteoDetect, and a Software-as-a-Service (SaaS) application, Glooko, both of which use AI to advise on results outcomes.12
AI can also support existing laboratory procedures. For example, it can serve as a supplementary or a validation tool in imaging analytics and help process more slides or culture plates in a shorter duration.
References
- Yao L, Wang L, Huang F, et al. A microfluidic impedance biosensor based on immunomagnetic separation and urease catalysis for continuous-flow detection of E. coli O157:H7. Sensor Actuat B-Chem. 2018;259:1013–21
- Qin, N., Zhao, P., Ho, E. et al Microfluidic Technology for Antibacterial Resistance Study and Antibiotic Susceptibility Testing: Review and Perspective. ACS Sens. 2021, 6, 3–21
- Bae E, Aroonnual A, Bhunia A, et al System automation for a bacterial colony detection and identification instrument via forward scattering. Meas Sci Technol. 2009,20:015802. doi:10.1088/0957-0233/20/1/015802
- Anders, H.J., Mannle, D., Carpenter, W., et al Multisite Qualification of an Automated Incubator and Colony Counter for Environmental and Bioburden Applications in Pharmaceutical Microbiology. PDA Journal of Pharmaceutical Science and Technology, 2022. DOI: 10.5731/pdajpst.2022.012742
- Sandle, T. (2018) Automated, Digital Colony Counting: Qualification and Data Integrity, Journal of GxP Compliance, 22 (1): 1-10
- Shi, J., Zhang, F., Wu, S. et al. Noise-free microbial colony counting method based on hyperspectral features of agar plates, Food Chemistry, 2019, 274: 925-932
- Sandle, T. Microbiological Identification with MALDI-TOF MS, Journal of Validation Technology, 2015, 21 (3): 1-10: https://www.researchgate.net/publication/284347366_Microbiological_Identification_with_MALDI-TOF_MS
- Cheng, J. and Xie, X. Vibrational spectroscopic imaging of living systems: An emerging platform for biology and medicine, Science, 2015, 350, aaa8870
- Rho, E., Kim, M., Cho, S. et al Separation-free bacterial identification in arbitrary media via deep neural network-based SERS analysis. Biosensors and Bioelectronics, 2022; 202: 113991 DOI: 10.1016/j.bios.2022.113991
- Heinle, C., Gaultier, N., Miller, D. et al MetaLIMS, a simple open-source laboratory information management system for small metagenomic labs. Gigascience, 2017, 6(6), p.gix025.
- Li, J., Wang, Z., Tao, G. et al Smart heightened-containment biological laboratory: Technologies, modules, and aims, Journal of Biosafety and Biosecurity, 2022, 4 (2): 89-97
- Sandle, T. (2018) Q&A: How AI can improve chronic conditions, Digital Journal at: https://www.digitaljournal.com/life/health/q-a-how-ai-can-improve-chronic-conditions/article/530685
About The Author:
Tim Sandle, Ph.D., is a pharmaceutical professional with wide experience in microbiology and quality assurance. He is the author of more than 30 books relating to pharmaceuticals, healthcare, and life sciences, as well as over 170 peer-reviewed papers and some 500 technical articles. Sandle has presented at over 200 events and he currently works at Bio Products Laboratory Ltd. (BPL), and he is a visiting professor at the University of Manchester and University College London, as well as a consultant to the pharmaceutical industry. Visit his microbiology website at https://www.pharmamicroresources.com.